Researchers from the department presented machine learning and artificial intelligence research at the thirty-sixth Conference on Neural Information Processing Systems (NeurIPS 2023).
Outstanding Dataset Paper
ClimSim: An Open Large-Scale Dataset For Training High-Resolution Physics Emulators In Hybrid Multi-Scale Climate Models
Sungduk Yu, Walter Hannah, Liran Peng, Jerry Lin, Mohamed Aziz Bhouri, Ritwik Gupta, Björn Lütjens, Justus C. Will, Gunnar Behrens, Nora Loose, Charles Stern, Tom Beucler, Bryce Harrop, Benjamin Hillman, Andrea Jenney, Savannah L. Ferretti, Nana Liu, Animashree Anandkumar, Noah Brenowitz, Veronika Eyring, Nicholas Geneva, Pierre Gentine, Stephan Mandt, Jaideep Pathak, Akshay Subramaniam, Carl Vondrick, Rose Yu, Laure Zanna, Ryan Abernathey, Fiaz Ahmed, David Bader, Pierre Baldi, Elizabeth Barnes, Christopher Bretherton, Julius Busecke, Peter Caldwell, Wayne Chuang, Yilun Han, YU HUANG, Fernando Iglesias-Suarez, Sanket Jantre, Karthik Kashinath, Marat Khairoutdinov, Thorsten Kurth, Nicholas Lutsko, Po-Lun Ma, Griffin Mooers, J. David Neelin, David Randall, Sara Shamekh, Mark Taylor, Nathan Urban, Janni Yuval, Guang Zhang, Tian Zheng, Mike Pritchard
Abstract:
Modern climate projections lack adequate spatial and temporal resolution due to computational constraints. A consequence is inaccurate and imprecise predictions of critical processes such as storms. Hybrid methods that combine physics with machine learning (ML) have introduced a new generation of higher fidelity climate simulators that can sidestep Moore’s Law by outsourcing compute-hungry, short, high-resolution simulations to ML emulators. However, this hybrid ML-physics simulation approach requires domain-specific treatment and has been inaccessible to ML experts because of lack of training data and relevant, easy-to-use workflows. We present ClimSim, the largest-ever dataset designed for hybrid ML-physics research. It comprises multi-scale climate simulations, developed by a consortium of climate scientists and ML researchers. It consists of 5.7 billion pairs of multivariate input and output vectors that isolate the influence of locally-nested, high-resolution, high-fidelity physics on a host climate simulator’s macro-scale physical state. The dataset is global in coverage, spans multiple years at high sampling frequency, and is designed such that resulting emulators are compatible with downstream coupling into operational climate simulators. We implement a range of deterministic and stochastic regression baselines to highlight the ML challenges and their scoring. The data (https://huggingface.co/datasets/LEAP/ClimSim_high-res) and code (https://leap-stc.github.io/ClimSim) are released openly to support the development of hybrid ML-physics and high-fidelity climate simulations for the benefit of science and society.
Objaverse-XL: A Colossal Universe of 3D Objects
Matt Deitke, Ruoshi Liu, Matthew Wallingford, Huong Ngo, Oscar Michel, Aditya Kusupati, Alan Fan, Christian Laforte, Vikram Voleti, Samir Yitzhak Gadre, Eli VanderBilt, Aniruddha Kembhavi, Carl Vondrick, Georgia Gkioxari, Kiana Ehsani, Ludwig Schmidt, Ali Farhadi
Abstract:
Natural language processing and 2D vision models have attained remarkable proficiency on many tasks primarily by escalating the scale of training data. However, 3D vision tasks have not seen the same progress, in part due to the challenges of acquiring high-quality 3D data. In this work, we present Objaverse-XL, a dataset of over 10 million 3D objects. Our dataset comprises deduplicated 3D objects from a diverse set of sources, including manually designed objects, photogrammetry scans of landmarks and everyday items, and professional scans of historic and antique artifacts. Representing the largest scale and diversity in the realm of 3D datasets, Objaverse-XL enables significant new possibilities for 3D vision. Our experiments demonstrate the improvements enabled with the scale provided by Objaverse-XL. We show that by training Zero123 on novel view synthesis, utilizing over 100 million multi-view rendered images, we achieve strong zero-shot generalization abilities. We hope that releasing Objaverse-XL will enable further innovations in the field of 3D vision at scale.
Causal discovery from observational and interventional data across multiple environments
Adam Li, Amin Jaber, Elias Bareinboim
Abstract:
A fundamental problem in many sciences is the learning of causal structure underlying a system, typically through observation and experimentation. Commonly, one even collects data across multiple domains, such as gene sequencing from different labs, or neural recordings from different species. Although there exist methods for learning the equivalence class of causal diagrams from observational and experimental data, they are meant to operate in a single domain. In this paper, we develop a fundamental approach to structure learning in non-Markovian systems (i.e. when there exist latent confounders) leveraging observational and interventional data collected from multiple domains. Specifically, we start by showing that learning from observational data in multiple domains is equivalent to learning from interventional data with unknown targets in a single domain. But there are also subtleties when considering observational and experimental data. Using causal invariances derived from do-calculus, we define a property called S-Markov that connects interventional distributions from multiple-domains to graphical criterion on a selection diagram. Leveraging the S-Markov property, we introduce a new constraint-based causal discovery algorithm, S-FCI, that can learn from observational and interventional data from different domains. We prove that the algorithm is sound and subsumes existing constraint-based causal discovery algorithms.
A Causal Framework for Decomposing Spurious Variations
Drago Plecko, Elias Bareinboim
Abstract:
One of the fundamental challenges found throughout the data sciences is to explain why things happen in specific ways, or through which mechanisms a certain variable X exerts influences over another variable Y. In statistics and machine learning, significant efforts have been put into developing machinery to estimate correlations across variables efficiently. In causal inference, a large body of literature is concerned with the decomposition of causal effects under the rubric of mediation analysis. However, many variations are spurious in nature, including different phenomena throughout the applied sciences. Despite the statistical power to estimate correlations and the identification power to decompose causal effects, there is still little understanding of the properties of spurious associations and how they can be decomposed in terms of the underlying causal mechanisms. In this manuscript, we develop formal tools for decomposing spurious variations in both Markovian and Semi-Markovian models. We prove the first results that allow a non-parametric decomposition of spurious effects and provide sufficient conditions for the identification of such decompositions. The described approach has several applications, ranging from explainable and fair AI to questions in epidemiology and medicine, and we empirically demonstrate its use on a real-world dataset.
Nonparametric Identifiability of Causal Representations from Unknown Interventions
Julius von Kügelgen, Michel Besserve, Liang Wendong, Luigi Gresele, Armin Kekić, Elias Bareinboim, David Blei, Bernhard Schölkopf
Abstract:
We study causal representation learning, the task of inferring latent causal variables and their causal relations from high-dimensional mixtures of the variables. Prior work relies on weak supervision, in the form of counterfactual pre- and post-intervention views or temporal structure; places restrictive assumptions, such as linearity, on the mixing function or latent causal model; or requires partial knowledge of the generative process, such as the causal graph or intervention targets. We instead consider the general setting in which both the causal model and the mixing function are nonparametric. The learning signal takes the form of multiple datasets, or environments, arising from unknown interventions in the underlying causal model. Our goal is to identify both the ground truth latents and their causal graph up to a set of ambiguities which we show to be irresolvable from interventional data. We study the fundamental setting of two causal variables and prove that the observational distribution and one perfect intervention per node suffice for identifiability, subject to a genericity condition. This condition rules out spurious solutions that involve fine-tuning of the intervened and observational distributions, mirroring similar conditions for nonlinear cause-effect inference. For an arbitrary number of variables, we show that at least one pair of distinct perfect interventional domains per node guarantees identifiability. Further, we demonstrate that the strengths of causal influences among the latent variables are preserved by all equivalent solutions, rendering the inferred representation appropriate for drawing causal conclusions from new data. Our study provides the first identifiability results for the general nonparametric setting with unknown interventions, and elucidates what is possible and impossible for causal representation learning without more direct supervision.
Estimating Causal Effects Identifiable from Combination of Observations and Experiments
Yonghan Jung, Ivan Diaz, Jin Tian, Elias Bareinboim
Abstract:
Learning cause and effect relations is arguably one of the central challenges found throughout the data sciences. Formally, determining whether a collection of observational and interventional distributions can be combined to learn a target causal relation is known as the problem of generalized identification (or g-identification) [Lee et al., 2019]. Although g-identification has been well understood and solved in theory, it turns out to be challenging to apply these results in practice, in particular when considering the estimation of the target distribution from finite samples. In this paper, we develop a new, general estimator that exhibits multiply robustness properties for g-identifiable causal functionals. Specifically, we show that any g-identifiable causal effect can be expressed as a function of generalized multioutcome sequential back-door adjustments that are amenable to estimation. We then construct a corresponding estimator for the g-identification expression that exhibits robustness properties to bias. We analyze the asymptotic convergence properties of the estimator. Finally, we illustrate the use of the proposed estimator in experimental studies. Simulation results corroborate the theory.
Causal Fairness for Outcome Control
Drago Plecko, Elias Bareinboim
Abstract:
As society transitions towards an AI-based decision-making infrastructure, an ever-increasing number of decisions once under control of humans are now delegated to automated systems. Even though such developments make various parts of society more efficient, a large body of evidence suggests that a great deal of care needs to be taken to make such automated decision-making systems fair and equitable, namely, taking into account sensitive attributes such as gender, race, and religion. In this paper, we study a specific decision-making task called outcome control in which an automated system aims to optimize an outcome variable Y while being fair and equitable. The interest in such a setting ranges from interventions related to criminal justice and welfare, all the way to clinical decision-making and public health. In this paper, we first analyze through causal lenses the notion of benefit, which captures how much a specific individual would benefit from a positive decision, counterfactually speaking, when contrasted with an alternative, negative one. We introduce the notion of benefit fairness, which can be seen as the minimal fairness requirement in decision-making, and develop an algorithm for satisfying it. We then note that the benefit itself may be influenced by the protected attribute, and propose causal tools which can be used to analyze this. Finally, if some of the variations of the protected attribute in the benefit are considered as discriminatory, the notion of benefit fairness may need to be strengthened, which leads us to articulating a notion of causal benefit fairness. Using this notion, we develop a new optimization procedure capable of maximizing Y while ascertaining causal fairness in the decision process.
Distribution-Free Statistical Dispersion Control for Societal Applications
Zhun Deng, Thomas Zollo, Jake Snell, Toniann Pitassi, Richard Zemel
Abstract:
Explicit finite-sample statistical guarantees on model performance are an important ingredient in responsible machine learning. Previous work has focused mainly on bounding either the expected loss of a predictor or the probability that an individual prediction will incur a loss value in a specified range. However, for many high-stakes applications, it is crucial to understand and control the dispersion of a loss distribution, or the extent to which different members of a population experience unequal effects of algorithmic decisions. We initiate the study of distribution-free control of statistical dispersion measures with societal implications and propose a simple yet flexible framework that allows us to handle a much richer class of statistical functionals beyond previous work. Our methods are verified through experiments in toxic comment detection, medical imaging, and film recommendation.
Representational Strengths and Limitations of Transformers
Clayton Sanford, Daniel Hsu, Matus Telgarsky
Abstract:
Attention layers, as commonly used in transformers, form the backbone of modern deep learning, yet there is no mathematical description of their benefits and deficiencies as compared with other architectures. In this work we establish both positive and negative results on the representation power of attention layers, with a focus on intrinsic complexity parameters such as width, depth, and embedding dimension. On the positive side, we present a sparse averaging task, where recurrent networks and feedforward networks all have complexity scaling polynomially in the input size, whereas transformers scale merely logarithmically in the input size; furthermore, we use the same construction to show the necessity and role of a large embedding dimension in a transformer. On the negative side, we present a triple detection task, where attention layers in turn have complexity scaling linearly in the input size; as this scenario seems rare in practice, we also present natural variants that can be efficiently solved by attention layers. The proof techniques emphasize the value of communication complexity in the analysis of transformers and related models, and the role of sparse averaging as a prototypical attention task, which even finds use in the analysis of triple detection.
Fast Attention Requires Bounded Entries
Josh Alman, Zhao Song
Abstract:
In modern machine learning, inner product attention computation is a fundamental task for training large language models such as Transformer, GPT-1, BERT, GPT-2, GPT-3 and ChatGPT. Formally, in this problem, one is given as input three matrices Q,K,V∈[−B,B]n×d, and the goal is to construct the matrix Att(Q,K,V):=diag(A1n)−1AV∈ℝn×d, where A=exp(QK⊤/d) is the `attention matrix’, and exp is applied entry-wise. Straightforward methods for this problem explicitly compute the n×n attention matrix A, and hence require time Ω(n2) even when d=no(1) is small.
In this paper, we investigate whether faster algorithms are possible by implicitly making use of the matrix A. We present two results, showing that there is a sharp transition at B=Θ(logn‾‾‾‾‾√).
∙ If d=O(logn) and B=o(logn‾‾‾‾‾√), there is an n1+o(1) time algorithm to approximate Att(Q,K,V) up to 1/poly(n) additive error.
∙ If d=O(logn) and B=Θ(logn‾‾‾‾‾√), assuming the Strong Exponential Time Hypothesis from fine-grained complexity theory, it is impossible to approximate Att(Q,K,V) up to 1/poly(n) additive error in truly subquadratic time n2−Ω(1).
This gives a theoretical explanation for the phenomenon observed in practice that attention computation is much more efficient when the input matrices have smaller entries.
Bypass Exponential Time Preprocessing: Fast Neural Network Training via Weight-Data Correlation Preprocessing
Josh Alman, Jiehao Liang, Zhao Song, Ruizhe Zhang, Danyang Zhuo
Abstract:
Over the last decade, deep neural networks have transformed our society, and they are already widely applied in various machine learning applications. State-of-art deep neural networks are becoming larger in size every year to deliver increasing model accuracy, and as a result, model training consumes substantial computing resources and will only consume more in the future. Using current training methods, in each iteration, to process a data point x∈ℝd in a layer, we need to spend Θ(md) time to evaluate all the m neurons in the layer. This means processing the entire layer takes Θ(nmd) time for n data points. Recent work [Song, Yang and Zhang, NeurIPS 2021] reduces this time per iteration to o(nmd), but requires exponential time to preprocess either the data or the neural network weights, making it unlikely to have practical usage.
In this work, we present a new preprocessing method that simply stores the weight-data correlation in a tree data structure in order to quickly, dynamically detect which neurons fire at each iteration. Our method requires only O(nmd) time in preprocessing and still achieves o(nmd) time per iteration. We complement our new algorithm with a lower bound, proving that assuming a popular conjecture from complexity theory, one could not substantially speed up our algorithm for dynamic detection of firing neurons.
Differentially Private Approximate Near Neighbor Counting in High Dimensions
Alexandr Andoni, Piotr Indyk, Sepideh Mahabadi, Shyam Narayanan
Abstract:
Range counting (e.g., counting the number of data points falling into a given query ball) under differential privacy has been studied extensively. However, the current algorithms for this problem are subject to the following dichotomy. One class of algorithms suffers from an additive error that is a fixed polynomial in the number of points. Another class of algorithms allows for polylogarithmic additive error, but the error grows exponentially in the dimension. To achieve the latter, the problem is relaxed to allow a “fuzzy” definition of the range boundary, e.g., a count of the points in a ball of radius r might also include points in a ball of radius cr for some c > 1.
In this paper, we present an efficient algorithm that offers a sweet spot between these two classes. The algorithm has an additive error that is an arbitrary small power of the data set size, depending on how fuzzy the range boundary is, as well as a small (1 + o(1)) multiplicative error. Crucially, the amount of noise added has no dependence on the dimension. Our algorithm introduces a variant of Locality-Sensitive Hashing, utilizing it in a novel manner.
Variational Inference with Gaussian Score Matching
Chirag Modi, Robert Gower, Charles Margossian, Yuling Yao, David Blei, Lawrence Saul
Abstract:
Variational inference (VI) is a method to approximate the computationally intractable posterior distributions that arise in Bayesian statistics. Typically, VI fits a simple parametric distribution to the target posterior by minimizing an appropriate objective such as the evidence lower bound (ELBO). In this work, we present a new approach to VI based on the principle of score matching, that if two distributions are equal then their score functions (i.e., gradients of the log density) are equal at every point on their support. With this, we develop score matching VI, an iterative algorithm that seeks to match the scores between the variational approximation and the exact posterior. At each iteration, score matching VI solves an inner optimization, one that minimally adjusts the current variational estimate to match the scores at a newly sampled value of the latent variables.
We show that when the variational family is a Gaussian, this inner optimization enjoys a closed form solution, which we call Gaussian score matching VI (GSM-VI). GSM-VI is also a “black box” variational algorithm in that it only requires a differentiable joint distribution, and as such it can be applied to a wide class of models. We compare GSM-VI to black box variational inference (BBVI), which has similar requirements but instead optimizes the ELBO. We study how GSM-VI behaves as a function of the problem dimensionality, the condition number of the target covariance matrix (when the target is Gaussian), and the degree of mismatch between the approximating and exact posterior distribution. We also study GSM-VI on a collection of real-world Bayesian inference problems from the posteriorDB database of datasets and models. In all of our studies we find that GSM-VI is faster than BBVI, but without sacrificing accuracy. It requires 10-100x fewer gradient evaluations to obtain a comparable quality of approximation.
Practical and Asymptotically Exact Conditional Sampling in Diffusion Models
Luhuan Wu, Brian Trippe, Christian Naesseth, David Blei, John Cunningham
Abstract:
Diffusion models have been successful on a range of conditional generation tasks including molecular design and text-to-image generation. However, these achievements have primarily depended on task-specific conditional training or error-prone heuristic approximations. Ideally, a conditional generation method should provide exact samples for a broad range of conditional distributions without requiring task-specific training. To this end, we introduce the Twisted Diffusion Sampler, or TDS. TDS is a sequential Monte Carlo (SMC) algorithm that targets the conditional distributions of diffusion models. The main idea is to use twisting, an SMC technique that enjoys good computational efficiency, to incorporate heuristic approximations without compromising asymptotic exactness. We first find in simulation and on MNIST image inpainting and class-conditional generation tasks that TDS provides a computational statistical trade-off, yielding more accurate approximations with many particles but with empirical improvements over heuristics with as few as two particles. We then turn to motif-scaffolding, a core task in protein design, using a TDS extension to Riemannian diffusion models. On benchmark test cases, TDS allows flexible conditioning criteria and often outperforms the state-of-the-art.
Causal-structure Driven Augmentations for Text OOD Generalization
Amir Feder, Yoav Wald, Claudia Shi, Suchi Saria, David Blei
Abstract:
The reliance of text classifiers on spurious correlations can lead to poor generalization at deployment, raising concerns about their use in safety-critical domains such as healthcare. In this work, we propose to use counterfactual data augmentation, guided by knowledge of the causal structure of the data, to simulate interventions on spurious features and to learn more robust text classifiers. We show that this strategy is appropriate in prediction problems where the label is spuriously correlated with an attribute. Under the assumptions of such problems, we discuss the favorable sample complexity of counterfactual data augmentation, compared to importance re-weighting. Pragmatically, we match examples using auxiliary data, based on diff-in-diff methodology, and use a large language model (LLM) to represent a conditional probability of text. Through extensive experimentation on learning caregiver-invariant predictors of clinical diagnoses from medical narratives and on semi-synthetic data, we demonstrate that our method for simulating interventions improves out-of-distribution (OOD) accuracy compared to baseline invariant learning algorithms.
Evaluating the Moral Beliefs Encoded in LLMs
Nino Scherrer, Claudia Shi, Amir Feder, David Blei
Abstract:
This paper presents a case study on the design, administration, post-processing, and evaluation of surveys on large language models (LLMs). It comprises two components: (1) A statistical method for eliciting beliefs encoded in LLMs. We introduce statistical measures and evaluation metrics that quantify the probability of an LLM “making a choice”, the associated uncertainty, and the consistency of that choice. (2) We apply this method to study what moral beliefs are encoded in different LLMs, especially in ambiguous cases where the right choice is not obvious. We design a large-scale survey comprising 680 high-ambiguity moral scenarios (e.g., “Should I tell a white lie?”) and 687 low-ambiguity moral scenarios (e.g., “Should I stop for a pedestrian on the road?”). Each scenario includes a description, two possible actions, and auxiliary labels indicating violated rules (e.g., “do not kill”). We administer the survey to 28 open- and closed-source LLMs. We find that (a) in unambiguous scenarios, most models “choose” actions that align with commonsense. In ambiguous cases, most models express uncertainty. (b) Some models are uncertain about choosing the commonsense action because their responses are sensitive to the question-wording. (c) Some models reflect clear preferences in ambiguous scenarios. Specifically, closed-source models tend to agree with each other.
The Distinguished Lecture series brings computer scientists to Columbia to discuss current issues and research that are affecting their particular research fields.
Cognitive Workforce Revolution with Trustworthy and Self-Learning Generative AI
Monica Lam, Stanford University
CS Auditorium (CSB 451)
November 15, 2023
11:40 AM to 12:40 PM
Generative AI, and in particular Large Language Models (LLMs), have already changed how we work and study. To truly transform the cognitive workforce however, LLMs need to be trustworthy so they can operate autonomously without human oversight. Unfortunately, language models are not grounded and have a tendency to hallucinate.
Our research hypothesis is that we can turn LLM into useful workers across different domains if we (1) teach them how to acquire and apply knowledge in external corpora such as written documents, knowledge bases, and APIs; (2) have them self-learn through model distillation of simulated conversations. We showed that by supplying different external corpora to our Genie assistant framework, we can readily create trustworthy agents that can converse about topics in open domains from Wikidata, Wikipedia, or StackExchange; help navigate services and products such as restaurants or online stores; persuade users to donate to charities; and improve the social skills of people with autism spectrum disorder.
Watch the Video of the Lecture
Causal Representation Learning and Optimal Intervention Design
Caroline Uhler, MIT
CS Auditorium (CSB 451)
November 8, 2023
11:40 AM to 12:40 PM
Massive data collection holds the promise of a better understanding of complex phenomena and, ultimately, of better decisions. Representation learning has become a key driver of deep learning applications since it allows learning latent spaces that capture important properties of the data without requiring any supervised annotations. While representation learning has been hugely successful in predictive tasks, it can fail miserably in causal tasks, including predicting the effect of an intervention. This calls for a marriage between representation learning and causal inference. An exciting opportunity in this regard stems from the growing availability of interventional data (in medicine, advertisement, education, etc.). However, these datasets are still minuscule compared to the action spaces of interest in these applications (e.g. interventions can take on continuous values like the dose of a drug or can be combinatorial as in combinatorial drug therapies). In this talk, we will present initial ideas towards building a statistical and computational framework for causal representation learning and discuss its applications to optimal intervention design in the context of drug design and single-cell biology.
Watch the Video of the Lecture
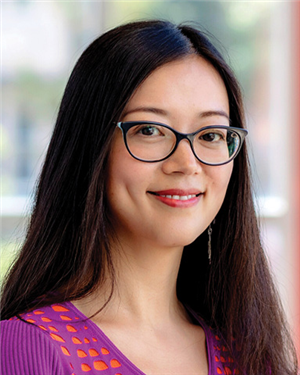
SmartBook: an AI Prophetess for Disaster Reporting and Forecasting
Heng Ji, University of Illinois at Urbana-Champaign
CS Auditorium (CSB 451)
November 1, 2023
11:40 AM to 12:40 PM
Abstract:
We propose SmartBook, a novel framework that cannot be solved by ChatGPT, targeting situation report generation which consumes large volumes of news data to produce a structured situation report with multiple hypotheses (claims) summarized and grounded with rich links to factual evidence by claim detection, fact checking, misinformation detection and factual error correction. Furthermore, SmartBook can also serve as a novel news event simulator, or an intelligent prophetess. Given “What-if” conditions and dimensions elicited from a domain expert user concerning a disaster scenario, SmartBook will induce schemas from historical events, and automatically generate a complex event graph along with a timeline of news articles that describe new simulated events based on a new Λ-shaped attention mask that can generate text with infinite length. By effectively simulating disaster scenarios in both event graph and natural language format, we expect SmartBook will greatly assist humanitarian workers and policymakers to exercise reality checks (what would the next disaster look like under these given conditions?), and thus better prevent and respond to future disasters.
Watch the Video of the Lecture
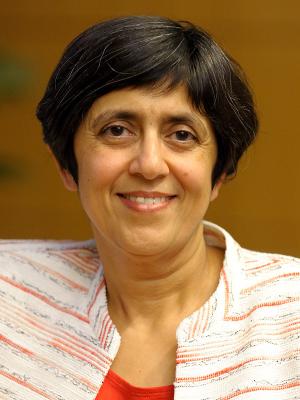
Enabling the Era of Immersive Computing
Sarita Adve, University of Illinois at Urbana-Champaign
CS Auditorium (CSB 451)
October 25, 2023
11:40 AM to 12:40 PM
Computing is on the brink of a new immersive era. Recent innovations in virtual/augmented/mixed reality (extended reality or XR) show the potential for a new immersive modality of computing that will transform most human activities and change how we design, program, and use computers. There is, however, an orders of magnitude gap between the power/performance/quality-of-experience attributes of current and desirable immersive systems. Bridging this gap requires an inter-disciplinary research agenda that spans end-user devices, edge, and cloud, is based on hardware-software-algorithm co-design, and is driven by end-to-end human-perceived quality of experience.
The ILLIXR (Illinois Extended Reality) project has developed an open source end-to-end XR system to enable such a research agenda. ILLIXR is being used in academia and industry to quantify the research challenges for desirable immersive experiences and provide solutions to address these challenges. To further push the interdisciplinary frontier for immersive computing, we recently established the IMMERSE center at Illinois to bring together research, education, and infrastructure activities in immersive technologies, applications, and human experience. This talk will give an overview of IMMERSE and a deeper dive into the ILLIXR project, including the ILLIXR infrastructure, its use to identify XR systems research challenges, and cross-system solutions to address several of these challenges.
Watch the Video of the Lecture
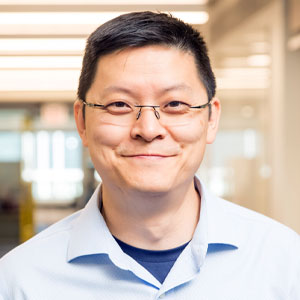
Protecting Human Users from Misused AI
Ben Zhao, University of Chicago
CS Auditorium (CSB 451)
October 9, 2023
11:40 AM to 12:40 PM
Abstract:
Recent developments in machine learning and artificial intelligence have taken nearly everyone by surprise. The arrival of arguably the most transformative wave of AI did not bring us smart cities full of self-driving cars, or robots that do our laundry and mow our lawns. Instead, it brought us over-confident token predictors that hallucinate, deepfake generators that produce realistic images and video, and ubiquitous surveillance. In this talk, I’ll describe some of our recent efforts to warn, and later defend against some of the darker side of AI.
In particular, I will tell the story of how our efforts to disrupt unauthorized facial recognition models led unexpectedly to Glaze, a tool to defend human artists against art mimicry by generative image models. I will share some of the ups and downs of implementing and deploying an adversarial ML tool to a global user base, and reflect on mistakes and lessons learned.
Watch the Video of the Lecture
The paper “I Want to Figure Things Out”: Supporting Exploration in Navigation for People with Visual Impairments” and three other papers from the Graphics & User Interfaces group will be presented at the 26th ACM Conference On Computer-Supported Cooperative Work And Social Computing (CSCW 2023).
“I Want to Figure Things Out”: Supporting Exploration in Navigation for People with Visual Impairments”
Gaurav Jain Columbia University, Yuanyang Teng Columbia University, Dong Heon Cho Columbia University, Yunhao Xing Columbia University, Maryam Aziz University of Connecticut, and Brian Smith Columbia University
Navigation assistance systems (NASs) aim to help visually impaired people (VIPs) navigate unfamiliar environments. Most of today’s NASs support VIPs via turn-by-turn navigation, but a growing body of work highlights the importance of exploration as well. It is unclear, however, how NASs should be designed to help VIPs explore unfamiliar environments. In this paper, we perform a qualitative study to understand VIPs’ information needs and challenges with respect to exploring unfamiliar environments to inform the design of NASs that support exploration. Our findings reveal the types of spatial information that VIPs need as well as factors that affect VIPs’ information preferences. We also discover specific challenges that VIPs face that future NASs can address, such as orientation and mobility education and collaborating effectively with others. We present design implications for NASs that support exploration, and we identify specific research opportunities and discuss open socio-technical challenges for making such NASs possible. We conclude by reflecting on our study procedure to inform future approaches in research on ethical considerations that may be adopted while interacting with the broader VIP community.
Social Wormholes: Exploring Preferences and Opportunities for Distributed and Physically-Grounded Social Connections
Joanne Leong MIT Media Lab, Yuanyang Teng Columbia University, Xingyu Liu University of California Los Angeles, Hanseul Jun Stanford University, Sven Kratz Snap, Inc., Yu Jiang Tham Snap, Inc., Andrés Monroy-Hernández Snap, Inc. and Princeton University, Brian Smith Snap, Inc. and Columbia University, and Rajan Vaish Snap, Inc.
Ubiquitous computing encapsulates the idea for technology to be interwoven into the fabric of everyday life. As computing blends into everyday physical artifacts, powerful opportunities open up for social connection. Prior connected media objects span a broad spectrum of design combinations. Such diversity suggests that people have varying needs and preferences for staying connected to one another. However, since these designs have largely been studied in isolation, we do not have a holistic understanding around how people would configure and behave within a ubiquitous social ecosystem of physically-grounded artifacts. In this paper, we create a technology probe called Social Wormholes, that lets people configure their own home ecosystem of connected artifacts. Through a field study with 24 participants, we report on patterns of behaviors that emerged naturally in the context of their daily lives and shine a light on how ubiquitous computing could be leveraged for social computing.
Exploring Immersive Interpersonal Communication via AR
Kyungjun Lee University of Maryland, College Park, Hong Li Snap, Inc.,
Muhammad Rizky Wellytanto University of Illinois at Urbana-Champaign, Yu Jiang Tham Snap, Inc., Andrés Monroy-Hernández Snap, Inc. and Princeton University, Fannie Liu Snap, Inc. and JPMorgan Chase, Brian A. Smith Snap, Inc. and Columbia University, Rajan Vaish Snap, Inc.
A central challenge of social computing research is to enable people to communicate expressively with each other remotely. Augmented reality has great promise for expressive communication since it enables communication beyond texts and photos and towards immersive experiences rendered in recipients’ physical environments. Little research, however, has explored AR’s potential for everyday interpersonal communication. In this work, we prototype an AR messaging system, ARwand, to understand people’s behaviors and perceptions around communicating with friends via AR messaging. We present our findings under four themes observed from a user study with 24 participants, including the types of immersive messages people choose to send to each other, which factors contribute to a sense of immersiveness, and what concerns arise over this new form of messaging. We discuss important implications of our findings on the design of future immersive communication systems.
Perspectives from Naive Participants and Social Scientists on Addressing Embodiment in a Virtual Cyberball Task
Tao Long Cornell University and Columbia University, Swati Pandita Cornell University and California Institute of Technology, Andrea Stevenson Won Cornell University
We describe the design of an immersive virtual Cyberball task that included avatar customization, and user feedback on this design. We first created a prototype of an avatar customization template and added it to a Cyberball prototype built in the Unity3D game engine. Then, we conducted in-depth user testing and feedback sessions with 15 Cyberball stakeholders: five naive participants with no prior knowledge of Cyberball and ten experienced researchers with extensive experience using the Cyberball paradigm. We report the divergent perspectives of the two groups on the following design insights; designing for intuitive use, inclusivity, and realistic experiences versus minimalism. Participant responses shed light on how system design problems may contribute to or perpetuate negative experiences when customizing avatars. They also demonstrate the value of considering multiple stakeholders’ feedback in the design process for virtual reality, presenting a more comprehensive view in designing future Cyberball prototypes and interactive systems for social science research.
Research papers from the Computer Vision Group were accepted to the International Conference on Computer Vision (ICCV ’23), the premiere international conference that includes computer vision workshops and tutorials.
ViperGPT: Visual Inference via Python Execution for Reasoning
Dídac Surís Columbia University, Sachit Menon Columbia University, Carl Vondrick Columbia University
Answering visual queries is a complex task that requires both visual processing and reasoning. End-to-end models, the dominant approach for this task, do not explicitly differentiate between the two, limiting interpretability and generalization. Learning modular programs presents a promising alternative, but has proven challenging due to the difficulty of learning both the programs and modules simultaneously. We introduce ViperGPT, a framework that leverages code-generation models to compose vision-and-language models into subroutines to produce a result for any query. ViperGPT utilizes a provided API to access the available modules, and composes them by generating Python code that is later executed. This simple approach requires no further training, and achieves state-of-the-art results across various complex visual tasks.
Zero-1-to-3: Zero-shot One Image to 3D Object
Ruoshi Liu Columbia University, Rundi Wu Columbia University, Basile Van Hoorick Columbia University, Pavel Tokmakov Toyota Research Institute, Sergey Zakharov Toyota Research Institute, Carl Vondrick Columbia University
We introduce Zero-1-to-3, a framework for changing the camera viewpoint of an object given just a single RGB image. To perform novel view synthesis in this under-constrained setting, we capitalize on the geometric priors that large-scale diffusion models learn about natural images. Our conditional diffusion model uses a synthetic dataset to learn controls of the relative camera viewpoint, which allow new images to be generated of the same object under a specified camera transformation. Even though it is trained on a synthetic dataset, our model retains a strong zero-shot generalization ability to out-of-distribution datasets as well as in-the-wild images, including impressionist paintings. Our viewpoint-conditioned diffusion approach can further be used for the task of 3D reconstruction from a single image. Qualitative and quantitative experiments show that our method significantly outperforms state-of-the-art single-view 3D reconstruction and novel view synthesis models by leveraging Internet-scale pre-training.
Muscles in Action
Mia Chiquier Columbia University, Carl Vondrick Columbia University
Human motion is created by, and constrained by, our muscles. We take a first step at building computer vision methods that represent the internal muscle activity that causes motion. We present a new dataset, Muscles in Action (MIA), to learn to incorporate muscle activity into human motion representations. The dataset consists of 12.5 hours of synchronized video and surface electromyography (sEMG) data of 10 subjects performing various exercises. Using this dataset, we learn a bidirectional representation that predicts muscle activation from video, and conversely, reconstructs motion from muscle activation. We evaluate our model on in-distribution subjects and exercises, as well as on out-of-distribution subjects and exercises. We demonstrate how advances in modeling both modalities jointly can serve as conditioning for muscularly consistent motion generation. Putting muscles into computer vision systems will enable richer models of virtual humans, with applications in sports, fitness, and AR/VR.
SurfsUp: Learning Fluid Simulation for Novel Surfaces
Arjun Mani Columbia University, Ishaan Preetam Chandratreya Columbia University, Elliot Creager University of Toronto, Carl Vondrick Columbia University, Richard Zemel Columbia University
Modeling the mechanics of fluid in complex scenes is vital to applications in design, graphics, and robotics. Learning-based methods provide fast and differentiable fluid simulators, however most prior work is unable to accurately model how fluids interact with genuinely novel surfaces not seen during training. We introduce SURFSUP, a framework that represents objects implicitly using signed distance functions (SDFs), rather than an explicit representation of meshes or particles. This continuous representation of geometry enables more accurate simulation of fluid-object interactions over long time periods while simultaneously making computation more efficient. Moreover, SURFSUP trained on simple shape primitives generalizes considerably out-of-distribution, even to complex real-world scenes and objects. Finally, we show we can invert our model to design simple objects to manipulate fluid flow.
Landscape Learning for Neural Network Inversion
Ruoshi Liu Columbia University, Chengzhi Mao Columbia University, Purva Tendulkar Columbia University, Hao Wang Rutgers University, Carl Vondrick Columbia University
Many machine learning methods operate by inverting a neural network at inference time, which has become a popular technique for solving inverse problems in computer vision, robotics, and graphics. However, these methods often involve gradient descent through a highly non-convex loss landscape, causing the optimization process to be unstable and slow. We introduce a method that learns a loss landscape where gradient descent is efficient, bringing massive improvement and acceleration to the inversion process. We demonstrate this advantage on a number of methods for both generative and discriminative tasks, including GAN inversion, adversarial defense, and 3D human pose reconstruction.
Four papers from the Wu Lab were presented at the 49th International Conference on Very Large Data Bases (VLDB 2023). VLDB features research talks, tutorials, demonstrations, and workshops on issues in data management, database, and information systems research.
JoinBoost: Grow Trees Over Normalized Data Using Only SQL
Zezhou Huang, Rathijit Sen, Jiaxiang Liu, Eugene Wu
Although dominant for tabular data, ML libraries that train tree models over normalized databases (e.g., LightGBM, XGBoost) require the data to be denormalized as a single table, materialized, and exported. This process is not scalable, slow, and poses security risks. In-DB ML aims to train models within DBMSes to avoid data movement and provide data governance. Rather than modify a DBMS to support In-DB ML, is it possible to offer competitive tree training performance to specialized ML libraries…with only SQL?
We present JoinBoost, a Python library that rewrites tree training algorithms over normalized databases into pure SQL. It is portable to any DBMS, offers performance competitive with specialized ML libraries, and scales with the underlying DBMS capabilities. JoinBoost extends prior work from both algorithmic and systems perspectives. Algorithmically, we support factorized gradient boosting, by updating theYvariable to the residual in the non-materialized join result. Although this view update problem is generally ambiguous, we identify addition-to-multiplication preserving, the key property of variance semi-ring to support rmse, the most widely used criterion. System-wise, we identify residual updates as a performance bottleneck. Such overhead can be natively minimized on columnar DBMSes by creating a new column of residual values and adding it as a projection. We validate this with two implementations on DuckDB, with no or minimal modifications to its internals for portability. Our experiment shows that JoinBoost is 3x (1.1x) faster for random forests (gradient boosting) compared to LightGBM, and over an order magnitude faster than state-of-the-art In-DB ML systems. Further, JoinBoost scales well beyond LightGBM in terms of the # features, DB size (TPC-DS SF=1000), and join graph complexity (galaxy schemas).
Saibot: A Differentially Private Data Search Platform
Zezhou Huang, Jiaxiang Liu, Daniel Alabi, Raul Castro Fernandez, Eugene Wu
Recent data search platforms use ML task-based utility measures rather than metadata-based keywords, to search large dataset corpora. Requesters submit a training dataset and these platforms search for augmentations (join or union compatible datasets) that, when used to augment the requester’s dataset, most improve model (e.g., linear regression) performance. Although effective, providers that manage personally identifiable data demand differential privacy (DP) guarantees before granting these platforms data access. Unfortunately, making data search differentially private is nontrivial, as a single search can involve training and evaluating datasets hundreds or thousands of times, quickly depleting privacy budgets.
We present Saibot, a differentially private data search platform that employs Factorized Privacy Mechanism (FPM), a novel DP mechanism, to calculate sufficient semi-ring statistics for ML over different combinations of datasets. These statistics are privatized once, and can be freely reused for the search. This allows Saibot to scale to arbitrary numbers of datasets and requests, while minimizing the amount that DP noise affects search results. We optimize the sensitivity of FPM for common augmentation operations, and analyze its properties with respect to linear regression. Specifically, we develop an unbiased estimator for many-to-many joins, prove its bounds, and develop an optimization to redistribute DP noise to minimize the impact on the model. Our evaluation on a real-world dataset corpus of 329 datasets demonstrates that Saibot can return augmentations that achieve model accuracy within 50 to 90% of non-private search, while the leading alternative DP mechanisms (TPM, APM, shuffling) are several orders of magnitude worse.
Pollock: A Data Loading Benchmark
Gerardo Vitagliano, Mazhar Hameed, Lan Jiang, Lucas Reisener, Eugene Wu, Felix Naumann
Any system at play in a data-driven project has a fundamental requirement: the ability to load data. The de-facto standard format to distribute and consume raw data is csv. Yet, the plain text and flexible nature of this format make such files often difficult to parse and correctly load their content, requiring cumbersome data preparation steps.
We propose a benchmark to assess the robustness of systems in loading data from non-standard csv formats and with structural inconsistencies. First, we formalize a model to describe the issues that affect real-world files and use it to derive a systematic “pollution” process to generate dialects for any given grammar. Our benchmark leverages the pollution framework for the csv format. To guide pollution, we have surveyed thousands of real-world, publicly available csv files, recording the problems we encountered. We demonstrate the applicability of our benchmark by testing and scoring 16 different systems: popular csv parsing frameworks, relational database tools, spreadsheet systems, and a data visualization tool.
ConnectorX: Accelerating Data Loading From Databases to Dataframes
Xiaoying Wang, Weiyuan Wu, Jinze Wu, Yizhou Chen, Nick Zrymiak, Changbo Qu, Lampros Flokas, George Chow, Jiannan Wang, Tianzheng Wang, Eugene Wu, Qingqing Zhou
Data is often stored in a database management system (DBMS) but dataframe libraries are widely used among data scientists. An important but challenging problem is how to bridge the gap between databases and dataframes. To solve this problem, we present ConnectorX, a client library that enables fast and memory-efficient data loading from various databases to different dataframes.
We first investigate why the loading process is slow and consumes large memory. We surprisingly find that the main overhead comes from the client-side rather than query execution or data transfer. We integrate several existing and new techniques to reduce the overhead and carefully design the system architecture and interface to make ConnectorX easy to extend to various databases and dataframes. Moreover, we propose server-side result partitioning that can be adopted by DBMSs in order to better support exporting data to data science tools. We conduct extensive experiments to evaluate ConnectorX and compare it with popular libraries. The results show that ConnectorX significantly outperforms existing solutions. ConnectorX is open sourced at: https://github.com/sfu-db/connector-x.
The group received the ISSTA 2023 Distinguished Paper Award for their paper, CONCORD: Clone-Aware Contrastive Learning for Source Code, a self-supervised pre-training strategy.
The department is extremely proud of all of our students! We honored this year’s graduates at a post-commencement event on May 17.
A number of students received awards from the department for their service and academic excellence. The list of CS awardees is in this year’s graduation handout.
University commencement was on May 17 and on May 15, the Columbia Engineering Class of 2023 gathered on the South Lawn of Columbia’s campus to celebrate Class Day.
CS students Ethan Wu and Julia Zhao share what they’ll remember from Columbia as they look ahead to the future.
CS students are among the grantees pursuing research-based master’s and doctoral degrees in the natural, social, and engineering sciences at US institutions.