Month: February 2018
When AI Steers Us Astray
Interview with Xi Chen, coauthor of Complexity Dichotomies for Counting Problems
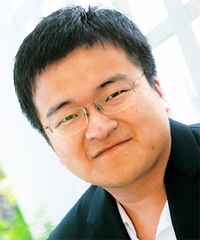
A counting problem asks the question: What is the exact number of solutions that exist for a given instance of a computational problem?
Theoretical computer scientists in the last 15 years have made tremendous progress in understanding the complexity of several families of counting problems, resulting in a number of intriguing dichotomies: a problem in each family is either easy—solvable in polynomial time—or computationally hard. Complexity Dichotomies for Counting Problems, Volume 1, Boolean Domain, a new book by Jin-Yi Cai (University of Wisconsin, Madison) and Xi Chen, provides a high-level overview of the current state of dichotomies for counting problems, pulling together and summarizing the major results and key papers that have contributed to progress in the field. This is the first time much of this material is available in book form.
Structured around several families of problems—counting constraint specification problems (#CSP), Holant, counting graph homomorphisms—the book begins by introducing many common techniques that are reused in later proofs. Each subsequent chapter revolves around a family of problems, starting with easier, specific cases before moving onto more complex and more general cases.
For the first volume, the authors focus on the Boolean domain (where variables are assigned 0-1 values); a second volume, expected to be released next year, will look at problems in the general domain where variables can be assigned three, four, or any number of values.
In this interview, Chen talks more about the motivation for the book and why dichotomies for counting problems is an area deserving of more study.
Why write the book?
Currently it’s hard for PhD students and others to get started in the field. Many of the papers are long and technically very dense, and they build on work done in previous papers. To understand one paper often requires a lot of backtracking. This can be frustrating at times because different papers use different notation and sometimes prove the same result or use the same tool in different contexts.
Our aim in writing this book was to minimize the effort needed to study dichotomies for counting problems. We summarize and streamline the major results using a common notation and unified framework so it is easier to see the progression of ideas and techniques. To encourage students to start working in this area, we wrote the book so an undergraduate with some basic knowledge of complexity theory can follow the proofs.
Could you define at a high level “dichotomies for counting problems”?
Counting problems involve determining the exact number of solutions that exist for a given input instance. Does a 3SAT instance have 10 or 11 satisfying assignments or 20 or 27? What is the exact number? In a graph, for instance, you might want to know the exact number of ways to apply three colors to vertices so that no two adjacent vertices share the same color.
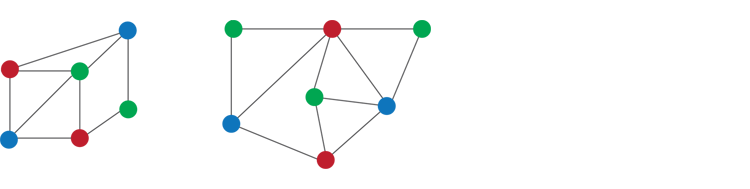
A number of families of counting problems have been studied. For example, we are interested in counting homomorphisms from an input graph G to a fixed graph H (where a homomorphism from G to H is a map f from vertices of G to vertices of H that preserves edges in G). In this case, each graph H defines a counting problem; e.g., when H is the triangle, the problem it defines is exactly the problem of counting 3-colorings. The 3-coloring problem is one of the classical problems known to be #P-hard, where #P is the complexity class that contains all counting problems, just as NP is for decision problems.
Can we classify EVERY graph H according to the complexity of the counting problem it defines? Such a result is called a dichotomy, which shows that all problems of a certain family can be classified into one of two groups: those admitting efficient algorithms and those that are #P-hard. Imagining each problem in a family as a dot, a dichotomy theorem can be viewed as a curve that classifies all the dots correctly according to their complexities. This curve typically involves a number of well-defined conditions: every problem that violates one of these conditions can be shown to be #P-hard and every problem that satisfies all the conditions can be solved by a unified efficient algorithm.

How has the field matured in the past few years?
Proofs have gotten stronger and more general. In the language of counting constraint satisfaction problems, the dichotomy theorem we had fifteen years ago only applied to problems defined by a single 0-1 valued symmetric function. In contrast we now have a full dichotomy for all counting constraint satisfaction problems (i.e., all problems defined by multiple complex-valued constraint functions with arbitrary arities).
What attracts you to the problem?
To me it is amazing that for these families of problems, a dichotomy theorem exists to once and for all classify the complexity of every problem. As it is shown by the classical Ladner’s theorem, there are infinitely many layers of problems in #P, ordered by their complexity, between P and #P-hard problems. Every time you move outside by one layer, problems become strictly harder.
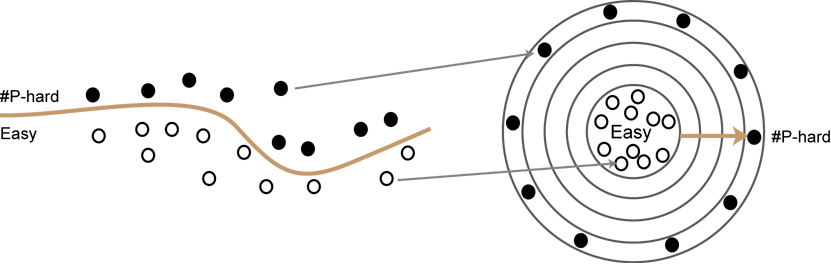
Dichotomy theorems covered in the book, however, show that all (infinitely many) problems in these lie either in P (the core) or the outmost #P-hard shell, and none of them belongs to layers in between. The intuition to me is that these families consist of “natural” counting problems only and do not contain any of those strange “#P-intermediate” problems, but I am curious: how much further can we go as we move to more and more general families of counting problems? Especially with Holant problems, not much is known for the general domain case. It’s one reason we need to develop stronger techniques to prove dichotomies for more general families of counting problems.
Volume 2 of Complexity Dichotomies for Counting Problems, which covers the general domain, is expected to be published next year.
Posted 02/21/18
Columbia team to compete in ACM-ICPC World Finals in Beijing
Three Columbia computer science graduate students have qualified to compete in the 2018 world finals of the Association for Computing Machinery (ACM) International Collegiate Programming Contest (ICPC) to be held April 15 – 20 in Beijing. The ACM-ICPC, sometimes called the “Olympics of Programming,” is the oldest and largest programming contest in the world and one of the most prestigious.
The students qualifying are Peilin Zhong, Ying Sheng, Haomin Long who, together with their coach Chengyu Lin, are all veterans of previous programming contests, including previous attempts to advance to the ICPC world finals.
The team, under the name CodeMbia, advanced this year after competing in the Greater New York Regional competition held November 19, where 52 area teams (including two other Columbia teams) were given five hours to complete 10 complex programming problems. CodeMbia solved 8 of the 10 problems, enough to tie with a Cornell team for first place, sending both onto the world finals.
With 2,948 universities competing in regional contests around the world, 128 teams earned the right to advance to the final round. Columbia last won a regional round in 2010, when the team that year traveled to Harbin, China, and finished 36th in the world.
Like in other programming contests, the ICPC regional problems consist of well-known, general computational problems that teams are asked to adapt for a specific case. Shortest path for instance might be presented as a subway map where competitors must determine the shortest path between two points. (The problems for the Greater New York Regional are given here.)
But the ICPC is a programming contest with a twist: three team members share a single computer.
“Other contests only measure how good you are in solving problems,” says Lin. “But the ICPC also tests how good you are at collaborating and at communicating with your team mates. So we have to practice collaboration, and how to divide up tasks to make the best use of the computer.”
With one person at the computer, the other two concentrate either on solving the problem or looking for errors or bugs, printing out the code to visually inspect it. Says Lin, “We have to learn to use the computer when it makes the most sense. We think a lot about how to solve the problem and how to verify that the algorithm works before we start coding. Otherwise, just coding for an hour is a waste of time.”
Hardest part for the team? “Not making mistakes. As contest veterans, we had the advantage of being able to solve the problems faster, so could spend more time double-checking our work for mistakes or for problems in the algorithm itself.”
The world finals will follow a similar format as the regionals—10 problems in five hours—but the problems themselves will be harder. So the team is stepping up its practice sessions, switching from the relatively easy regional problems to the more difficult ones from past finals.
The competition will be harder also. Facing CodeMbia will be 127 other qualifying teams. Lin makes no predictions for how well the team will do in the finals, but he stresses the fun of programming and looks forward to the challenge of competing against the world’s best collegiate programmers.
The ICPC world finals takes place at Peking University April 15 – 20.
Posted 2/20/18
– Linda Crane
3D Printing May Be the Key to Affordable Data Storage Using DNA
Donald F. Ferguson joins department as Professor of Practice
Donald F. Ferguson, after 30 years of technical and executive leadership in the computer industry, is joining Columbia’s Computer Science Department as Professor of Practice. He will be the first in the department to have the title.
For students, his appointment means a chance to learn from an industry insider the nuts and bolts of how computer science is practiced in the real world and how it differs from computer science as it is taught in a classroom.
“We learn things in industry the hard way,” says Ferguson. “I may not be able to teach it the right way, but I’ve done it all the different ways; by process of elimination, this has to be the right way because every other way I’ve done it has been wrong.”
Ferguson’s career started at IBM. For ten years he worked in IBM Research before a project of his got moved into production (becoming WebSphere), with him following to oversee architecture and technical innovation. From then on, he did less hands-on engineering and more “advise and consent,” that is, giving advice on design and then the OK to move a project forward. After being made chief architect for the Software Group at IBM, Ferguson was in charge of hundreds of products and thousands of engineers. Later at Microsoft, he managed projects exploring the future of enterprise software before moving to CA (formerly Computer Associates) where as CTO he led new product and technology development. In 2012, he moved to Dell, where as vice president and CTO for the company’s Software Group, he oversaw architecture, implementation design, technical strategy, and innovation.
Through his many positions at leading computer companies, Ferguson was directly involved in architecting numerous complex, end-to-end systems and services: hybrid cloud management, BYOD/mobility management, Internet-of-Things, and next-generation connected security. For his technical expertise and leadership, Ferguson holds the distinction of being the only person to be appointed an IBM Fellow, a Microsoft Technical Fellow, and a Dell Senior Fellow.
In his most recent role, he is an entrepreneur, having cofounded Seeka.TV, a streaming and social platform for connecting producers and consumers of video. Though nominally CTO, with only four in the company, there is no more advise and consent; he’s back to writing software.
He enjoys teaching also and for the past 10-15 years on and off, he has been an adjunct professor at Columbia, teaching advanced courses in serverless computing, cloud applications, modern as-a-service applications, and other topics directly related to his work experience. While teaching is for him an opportunity to learn, it has also given him insight into the huge gulf in how industry operates vs how software and programming are taught to students. Scale of programming is one issue—a piece of code written in a semester compared to 20M lines of code in a deployed application—but there are many others.
“The vast majority of the programming students learn is function call-return; wait a certain amount of time for a call back. But in industry, there are asynchronous applications and event-driven and queuing-based applications. Systems are big and complex, geographically distributed, and under different organizational control so it’s necessary to build and structure software that can accommodate all these different levels of complexity.”
“Don can draw from his invaluable and varied industry experience to offer great courses for our students,” says Julia Hirschberg, chair of the Computer Science Department. “We are delighted to have him returning to the department as Professor of Practice so he can teach many more students and offer more classes.”
Ferguson’s association with Columbia is of long standing. Columbia granted him a PhD in Computer Science (1989) for his thesis in applying economic models to manage system resources in distributed systems; he has taught at Columbia as an adjunct professor; and in 2013 the Columbia Engineering Alumni Association awarded him the Egleston Medal citing his contributions as a thought-leader in cloud computing, the father of WebSphere, and leader of software design.
Over his 30-year career, Ferguson has seen and been a part of tremendous technological change: from huge mainframes attended by a select coterie of engineers to computers hidden in light switches; from programming being something mysterious and little-understood to something done by high school students and others who may have little interest in a computer science degree; from all the computing power needed to send a man to the moon eclipsed by the power of an iPhone anyone can buy.
He laughs off an invitation to predict where technology will be in 10 years.
“One of the things that’s nice about being a college professor is teaching the people who will be deciding where things go in 10 years. I always tell students to stay in touch. I want to know what great things these students are going to be doing.”
This semester, Ferguson is teaching both Introduction to Databases (4111) and Introduction to Computing for Engineers and Applied Scientists (1006).
Posted 02/13/2018
– Linda Crane
Paper coauthored by Eugene Agichtein earns test-of-time award at WSDM 2018
“Finding High-Quality Content in Social Media” was originally
presented at the 2008 ACM International Conference on Web Search and Data
Mining (WSDM). Agichtein (PhD ’05, advisor: Luis Gravano) is
now Associate Professor at Emory University.
Three faculty promoted to Associate Professor
Augustin Chaintreau, Yaniv Erlich, and Daniel Hsu have been promoted from Assistant to Associate Professor without tenure.
Augustin Chaintreau
Augustin Chaintreau is interested in personal data and what happens to it. Who is using it and for what purposes? Is personalization giving everyone a fair chance? These are not questions Facebook, Google, or other web service companies necessarily want to answer, but large-scale, unchecked data mining of people’s personal data poses multiple risks.
To understand and mitigate these risks, Chaintreau is using mathematical analyses of networks and statistical methods to build tools and models that track how data flows, or predict how user behaviors affect them. Using those techniques, we can learn for instance why someone using online services is presented with certain ads and not others, or how a keyword (“sad”) typed in an email or an online search triggers an ad about depression or shamanic healing. Or we can prove that graph-based recommendation reinforces historical prejudice.
Connections between user actions across apps, captured and distilled over complex networks, are generally not known to people using the web. In exposing the downsides of personalization and data sharing, Chaintreau’s research often makes headlines. The New York Times, Washington Post, Economist, and Guardian have all reported on various aspects of his research, such as when he showed how geotagged data from only two apps is enough to identify someone, and that 60% of people will forward a link without reading it.
Chaintreau’s intent is not to prevent data sharing—he sees the benefits for health, energy efficiency, and public policy—but to bring transparency and accountability to the web so people can safely manage and share their personal data. Tools may inform and empower people, keep providers accountable, and sometimes present simple new ways to improve fairness.
An active member of the network and web research community, Chaintreau chaired the conference and program committees of ACM SIGMETRICS, ACM CoNEXT, and the annual meeting of the Data Transparency Lab. He served on the program committees of ACM SIGMETRICS, SIGCOMM, WWW, EC, CoNEXT, MobiCom, MobiHoc, IMC, WSDM, COSN, AAAI ICWSM, and IEEE Infocom, and is currently the founding editor in chief of the Proceedings of the ACM POMACS Series, after editing roles for IEEE TMC, ACM SIGCOMM CCR, and ACM SIGMOBILE MC2R.
For significant contributions to analyzing emerging distributed digital and social networking systems, he received the 2013 ACM SIGMETRICS Rising Star Researcher Award and he earned an NSF CAREER Award also in 2013.
Yaniv Erlich
Working in the field of quantitative genomics, Yaniv Erlich is at the forefront of new gene sequencing techniques and the issue of genetic privacy.
He was among the first to flag the ethical complexities of genetic privacy. A paper he spearheaded while a fellow at MIT’s Whitehead Institute showed how easy it is to take apparently anonymized genetic information donated by research participants and cross-reference it to online data sources to obtain surname information. For this, Nature Journal gave him the title Genome Hacker.
Since coming to Columbia in 2015 (through a joint appointment with the NYC Genome Center), Erlich has kept up a fast pace of new research, creating new algorithms for examining genetic information at both the molecular level and within large-scale human populations. Among his projects: software that takes only minutes to verify someone’s identity from a DNA sample; a DNA storage strategy 60% more efficient than previous methods (and approaching 90% of the theoretical maximum amount of information per nucleotide); a finding that short tandem repeats, once thought to be neutral, play an important role in regulating gene expression. In addition, he cofounded DNA.land, a crowd-sourcing site where people can donate their genome data for scientific research. Last year, he was named Chief Scientific Officer at MyHeritage, where he leads scientific development and strategy.
In his teaching too he is integrating new genetics research. His computer science class Ubiquitous Genomics was among the first courses to incorporate portable DNA sequencing devices into the curriculum, allowing students to learn DNA sequencing by doing it themselves.
Erlich’s awards include the Burroughs Wellcome Career Award (2013), the Harold M. Weintraub Award (2010), DARPA’s Young Faculty Award (2017), and the IEEE/ACM-CS HPC Award (2008). He was also recognized as one of 2010 Tomorrow’s PIs team of Genome Technology.
Daniel Hsu
A central challenge in machine learning is to reliably and automatically discover hidden structure in data with minimal human intervention. Currently, however, machine learning relies heavily on humans to label large amounts of data, an expensive, time-consuming process that Daniel Hsu aims to streamline through new algorithmic approaches that also address the statistical difficulties of a problem. An interactive learning method he created as a PhD student selectively chooses a small set of data examples that it queries users to label. Applied to electrocardiograms, his method reduced the amount of training data by 90 percent.
There are both theoretical and applied aspects to Hsu’s research. As a theoretician, he has produced the first computationally efficient algorithms for several statistical estimation tasks (including many involving latent variable models such as mixture models, hidden Markov models, and topic models), provided new algorithmic frameworks for solving interactive machine learning problems, and led in the creation of scalable tools for machine learning applications.
On the application side, Hsu has contributed to methods used in web personalization, automated natural language processing, and greater transparency to how personal data is used on the web. More recently, his research helped understand the role of gene regulation in disease, infer properties of dark energy in the universe from weak gravitational lensing, and characterize complex materials within a nanoscale structure.
At Columbia since 2013, Hsu has been recognized with a Yahoo ACE Award (2014), selected as one of “AI’s 10 to Watch” in 2015 by IEEE Intelligent Systems, and received a 2016 Sloan Research Fellowship. Just last year, he was made a 2017 Kavli Fellow.
He is a prolific author (10 papers in 2017 alone) and a member of the Data Science Institute (DSI) and the DSI’s TRIPODS Institute, where he collaborates with others to advance machine learning by tying together techniques from statistics, computer science, and applied mathematics.
Posted 02/02/2018
– Linda Crane
New cryptocurrencies offer better anonymity, new security challenges
With Amazon Research Award, Eugene Wu will add interactivity and adversarial generation to entity matching
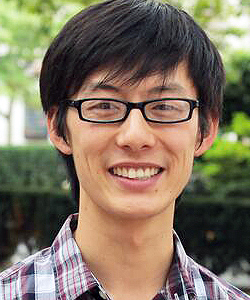
For his proposal “Interactive Matcher Debugging via Adversarial Generation,” Eugene Wu has been awarded an Amazon Research Award. This unrestricted gift, open to institutions of higher learning in North America and Europe, is intended to support one or two graduate students or post-doc researchers doing research under the guidance of the faculty member receiving the award.
An Assistant Professor of Computer Science at Columbia, Wu heads the WuLab research group and is a member of the Data Science Institute, where he co-chairs the Center for Data, Media and Society.
Wu will use the award to fund research that will extend existing techniques for entity matching, a data cleaning task that locates duplicate data entries referencing the same real-world entity (to learn, for example, that “M. S. Smith” and “Mike S. Smith” refer to the same person).
“Finding these duplicate records is crucial for integrating different data sources, and current methods rely on machine learning,” says Wu. “The problem is that machine learning is not perfect and it is hard to even anticipate where it is matching incorrectly. We are very pleased that Amazon has selected to fund this project where we will work toward two goals: help users identify cases likely to result in matching errors, and identify realistic data examples to use to improve the machine learning model.”
Online retailers and services collect their data from a huge number of different data sources that may use different spellings or naming conventions and provide different images or descriptions for the same item. Amazon might have hundreds of vendors listing and selling iPhones or iPhone-related products. If all of this data is uploaded to Amazon without being matched, customers might see 100 product pages for “iPhone,” “iPhone 6X Large,” “IPhONE 6 Best,” or even worse, a product search might come up empty if the search string doesn’t exactly match the product name as it is assigned by the vendor. Entity matching helps prevent these types of problems but entity matching is a deeply challenging problem.
Entity matching is a classification problem, with ensemble trees often used to label entity pairs as matching or non-matching. Errors in classification are a perennial problem, but if users understand why such errors occur, they can adjust the classification process to reduce errors.
Much of Wu’s research, and the focus of WuLab, has centered on creating interactive visualizations that make it easier for users to spot anomalous patterns and other problems while also providing explanations of why certain problems occur. Wu will do the same for entity matching by developing new algorithms and software components that, once inserted into existing entity-matching pipelines, will automatically generate high-level, easy-to-understand explanations so developers can readily understand what matching errors exist.
Once errors have been identified, the second direction of the research is to address the error. Wu will do so by generating training samples to feed back into the machine learning model and ultimately improve its accuracy. As with all classification tasks, entity matching requires huge amounts of training data, and it is often necessary to synthetically create additional data to train a classification model. One such data-generation technique is adversarial generation, which creates new data by taking a supplied, real-world string of values and changing one or two values to produce a new data string similar to real-world ones.
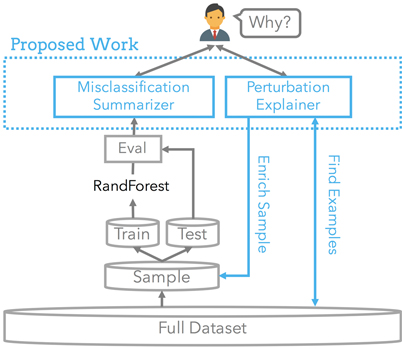
Widely used for images, adversarial generation has drawbacks for text since perturbing a single value often produces non-semantic text samples that wouldn’t actually occur in the real world; such data, inserted into the training set, could contribute to matching errors. Here, Wu proposes constraints that will consider the entire data record (not just individual string values) when perturbing transformations, thereby ensuring synthetic data is less arbitrary, more representative of real-world cases, and in the case of text, more semantically meaningful.
As part of the award, which totals $80K with an additional $20K in Amazon Web Services (AWS) Cloud Credits, Wu and his research group will meet and collaborate with Amazon research groups. The resulting paper will be published and the code written for the project will be made open source for other research groups.
Posted 02/01/2018
– Linda Crane