Five CS graduates awarded the Jonathan Gross Prize
The Jonathan Gross Prize honors students who graduate at the top of their class with a track record of promising innovative contributions to computer science. Five graduating computer science students—one senior from each of the four undergraduate schools and one student from the master’s program—were selected to receive the prize, which is named for Jonathan L. Gross, the popular professor emeritus who taught for 47 years at Columbia and cofounded the Computer Science Department. The prize is made possible by an endowment established by Yechiam (Y.Y.) Yemini, who for 31 years until his retirement in 2011, was a professor of computer science at Columbia.
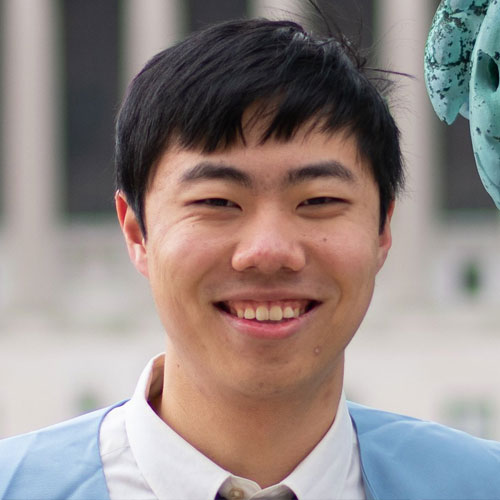
Steven Shao
Master of Science
I feel a deep appreciation for the potential of computers to express and realize complex ideas.
A childhood dream of building games for friends propelled Steven Shao into the world of programming and math. His interest in computer science remained with him when he first came to Columbia for undergrad and his appreciation of it has grown even more.
“Whenever I come across a technical idea in CS, like in the algorithms of ML, systems, or theory, there’s something irresistible about exploring and optimizing them further,” said Shao. “It’s like going down a rabbit hole of puzzles.”
He is inspired by how computers can be more human and how it affects society. Through classes, like computer vision, he learned how artificial intelligence powers the mechanisms underlying “this magic”. Shared Shao, “I wholly enjoyed professor Carl Vondrick’s lectures, starting from the long history of traditional vision and its relationship to optics and graphics, to its recent revolution with deep learning.”
Classes wrapped for Shao in December and he recently started as a software developer at Jane Street in downtown New York City.
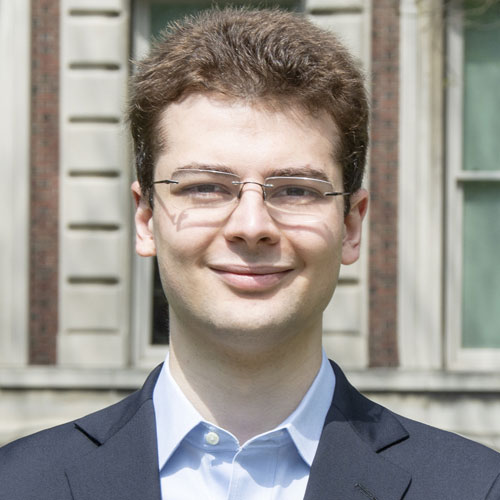
Alexandre Lamy
Columbia Engineering valedictorian
There are a few aspects that, in my mind, make computer science a unique and beautiful subject to study.
Alexandre Lamy loves the kind of logical thinking and problem solving approach that is needed in computer science to turn vague thoughts and ideas into precise algorithms. Each class he took showed him the beauty of the field and pushed him.
Machine learning with professor Nakul Verma was the class he liked the most. Said Lamy, “The class simultaneously gives you a deep understanding of the foundations of ML and teaches you most of the advanced mathematics you’ll need in more difficult classes – linear algebra, probability, and statistics concepts that are relevant to CS and not really covered in the corresponding required classes.” Lamy ended up working as a teacher’s assistant three times after taking the class and Verma became an important mentor for the rest of his time at Columbia.
Lamy looks forward to solving even more complex problems and formulating algorithms when he returns to Columbia in the fall for a MS in computer science.
“Looking around our world reveals an incredible number of real life problems that have been solved using computers,” he said. “But the amount of problems that are still out there and waiting to be solved seems to defy the imagination.”
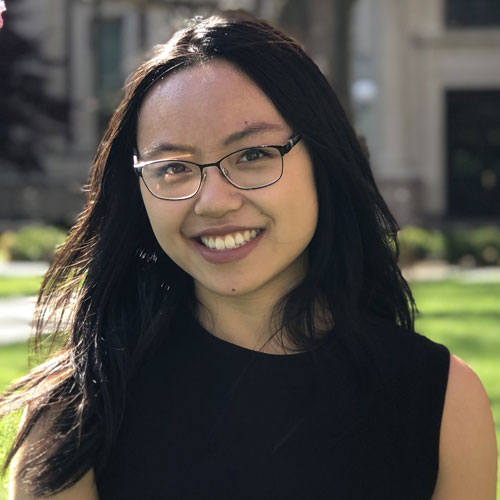
Linnie Jiang
Columbia College
I really appreciate how CS relies on a foundation of math so that results can be explained in a clean logical language that is familiar to us.
Computer science can be applied to so many domains and the interdisciplinarity of CS is what appeals to Jiang. While at Columbia her research with professors from the neuroscience department studied how expectation is encoded in the circuitry of the fruit fly (Drosophila melanogaster). Her computational background contributed to a deeper understanding of how experience-dependent learning is largely a circuit phenomenon driven by the interactions of an ensemble of neurons (aka a graph!).
“I was surprised by how continuous and unending research is—if you answer one question, the next immediately pops up,” said Jiang. “It seems like there are endless implications of every discovery you make.”
Jiang will pursue a PhD in Neurosciences at Stanford University, supported by funding from the Stanford Graduate Fellowship as well as the NSF Graduate Research Fellowship. She also plans to obtain a PhD minor in computer science while at Stanford.
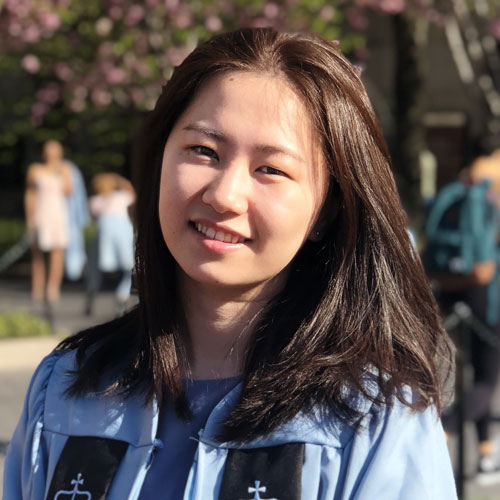
April Tang
Barnard College
Being a computer science major allowed me to combine everything I was interested in into one degree.
April Tang came to Barnard knowing that she would study mathematics or statistics, but an intro to Java class opened her up to computer science. She quickly realized she could combine math and statistics with CS because the structural thinking in object-oriented programing and CS theory aligned perfectly with her training from mathematics.
“I was even more amazed to find that I can use my statistics knowledge in real-world applications through the use of machine learning techniques,” said Tang.
That interest in machine learning led her to a natural language processing class. It is within the context of natural language processing where questions that might seem vague in other general machine learning courses become concrete problems. Tang shared, “Having the context in mind really motivates me and allows me to think in more depth about the pros and cons of each method.”
As a Barnard student, Tang took most of her liberal arts requirements at the school. One class she particularly adored was dance in New York City – where coursework was on analyzing the choreography of different dance performances.
In July, she will join Citigroup as a quantitative analyst in their New York office. Tang hopes to study data science and go back to school for a graduate degree in the future.
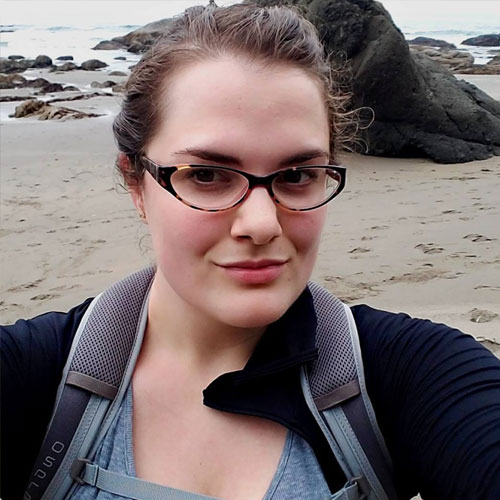
Rose Orenbuch
General Studies
When I took my first computer science course I realized that I would prefer an analytic and quantitative approach to biological questions.
Rose Orenbuch aspires to an academic career in the biosciences with a focus on genomics and neuroscience. She credits the skills she has gained from her CS courses as useful in conducting research – the ability to program and quickly learn new languages is particularly useful in bioinformatics, whether for statistical analysis or tool building or debugging other people’s programs.
A class that was both a favorite and the most challenging for her was computational genomics taught by professor Itsik Pe’er. Shared Orenbuch, “It was very helpful to understand the algorithms behind the tools I had been using in my research prior to the course.”
For that class, she built a tool for typing human leukocyte antigens (HLAs). HLAs are cell-surface proteins that are responsible for the regulation of the immune system. The tool has since been published and a paper describing it is currently under review at Bioinformatics.
During the summer, she will continue research to develop a tool to detect HLA expression imbalance and investigate its effect on outcomes of immunotherapy. Orenbuch is set to attend Harvard University for a PhD in systems biology in the fall.