Nakul Verma joins the department, bringing expertise and experience in machine learning
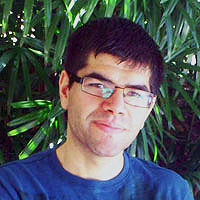
The number of computer science majors at Columbia is expected to increase yet again this year, driven in part by the exploding interest in machine learning. Among the 10 MS tracks, machine learning is by far the most popular, selected by 60% of the department’s master’s students (vs 12% for the second most popular).
According to Nakul Verma, who joins the department this semester as lecturer in discipline, this interest in machine learning is not likely to abate any time soon. “Machine learning is growing in popularity because it has so much applicability for fields outside computer science. Every application domain is incorporating machine learning techniques, and every traditional model is being challenged by the advent of big data.”
As a PhD student at UC San Diego, Verma gravitated toward machine learning’s theory side, what he sees as fruitful territory. “I love to learn new things, and machine learning theory has this ability to borrow ideas from other fields—mathematics, statistics, and even neuroscience. Borrowing ideas will continually grow machine learning as a field and it makes the field especially dynamic.”
Verma does his borrowing from differential geometry in mathematics, a field he had not previously studied in depth. But as machine learning shifts from strictly linear models to include nonlinear ones, new methods are needed to analyze and leverage intrinsic structure in data.
As examples of nonlinear data sets, Verma cites speech and articulations of robot motions, where data sets are high dimensional, containing many, many observations, each one associated with a potentially high number of features. However, relationships between data points may be fixed in some way so that a change in one causes a predictable change in another, giving the data an intrinsic structure. A robot might focus on key points in a gesture, analyzing how fingers move in relation to one another or to the wrist or arm. These movements are restricted along certain degrees of freedom—by joints, by the positioning of other fingers—suggesting the intrinsic structure of the data is in fact low-dimensional and that the xyz points of these joints form a manifold, or curved surface, in space.
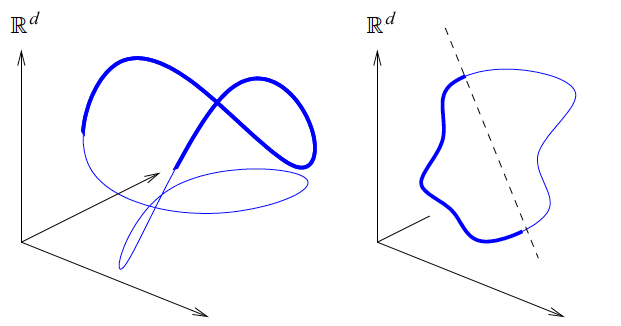
For a way to compress a manifold surface into lower dimensions without collapsing the underlying intrinsic structure, Verma looked to John Nash’s process for embedding manifolds, learning the math—or getting used to it—enough to understand how it could be applied to machine learning. Where Nash worked in terms of equations, Verma is working with actual data so the problem, laid out in Verma’s thesis and other papers by him, is to derive an algorithm from Nash’s technique, one that would work on today’s data sets.
While Verma’s thesis was highly theoretical, it had almost immediate practical applications. For four years at Janelia Research Campus HHMI, Verma helped geneticists and neuroscientists understand how genetics affects the brain to cause different behaviors. Working with fruit flies and other model organisms, researchers would modify certain genes thought to control aggression, social interactions, mating, and other behaviors and then record the organisms’ activity. The scale of data—from the thousands of modifications to the recorded video and audio imagery along with the neuronal recordings—was immense. Verma’s job was to tease out from the pile of data the small threads of how one change affects another, to pinpoint the relationships between the genetic modifications to the brain and the observed behavior. Verma’s work on manifolds and understanding intrinsic structure in data was crucial in developing practical yet statistically sound biological models.
Theory and application go hand in hand in machine learning, and the classes Verma will be teaching will contain a good dose of both, with the exact mix calibrated differently for grads vs undergrads. In either case, Verma sees a solid foundation on basic principles as necessary for understanding how a model is set up or why a certain framework is better than another. “The practical applications help reinforce the theory side of things. Teaching random forests should explain the basic theory but show also how it’s used in the real world. It’s not just some bookish knowledge; it’s one way Amazon and other companies reduce fraud.” Verma talks from experience, having worked at Amazon before going to Janelia.
But teaching has always been his ultimate goal. While at Janelia, Verma was awarded Teaching Fellowship, and last summer taught at Columbia as an adjunct. Says Verma, “Helping students achieve their goals and sharing their excitement for the subject is one of the most rewarding experiences of my academic career.”
Posted 10/02/2017
– Linda Crane