Genome-wide association studies, minus the Winner’s Curse, are shown to be reliable and replicable
The Winner’s Curse—where newly discovered genetic effects are consistently overestimated and fail consistently to replicate in subsequent studies—afflicts every genome-wide association study (GWAS). This failure to replicate calls into doubt the credibility of these studies that otherwise show promise in establishing the genetic basis for both quantitative (height, obesity) and disease traits. But how much of the failure to replicate is due to the Winner’s Curse? Are other factors involved? To find out, two Columbia University researchers analyzed 332 GWAS quantitative papers to de-bias the effects of the Winner’s Curse across a broad set of traits. Their first finding? Fully 70% of GWAS did not report enough information to independently verify replication rates. Digging deeper into papers that did fully report, the researchers identified two main issues preventing replication—use of populations with different ancestry, and not reporting a cohort size for each variant. Papers without these problems did in fact replicate at expected rates. Reporting deficiencies, not the paradigm of GWAS, are to blame for the failure to replicate. The researchers’ method and the code to implement the method are made available to other researchers in the field.
Large-scale genome-wide association studies (GWAS) scan the genomes of hundreds of thousands of individuals for genetic variations common to an entire population, allowing scientists to associate traits (height, risk of diabetes) to specific genetic variations, specifically single nucleotide polymorphisms, or SNPs (pronounced “snips”).
It’s a statistical approach that looks across a very large sampling of human genomes to discover what SNPs occur more frequently in individuals exhibiting the same traits, to see for instance, what SNPs are common in tall people or in those who are obese. That GWAS cost a fraction of full DNA sequencing have made GWAS especially attractive.
But are GWAS reliable? Associations found in a GWAS often do not replicate in subsequent studies assembled to corroborate such findings. GWAS are susceptible to the Winner’s Curse, where initial discoveries are too optimistic and fail to replicate more than expected. High failure rates in GWAS call into question the credibility of the GWAS paradigm.
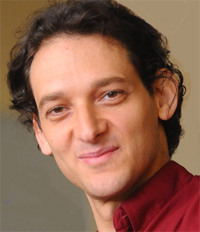
Itsik Pe’er, a computational geneticist and computer scientist at Columbia University, explains the term Winner’s Curse this way: “The millions of variants in the genome are all going through a statistical test. This is analogous to athletes competing in a time trial. The winner is very likely to be better than the average participant, but also likely to have been lucky, having had a particularly good day at the race. The time set by the winner therefore overestimates how good the winner is. Similarly, the statistical score of the winning variants overestimates the effect a winner has on the examined trait.”
A single GWAS can uncover thousands of SNPs; without the resources to investigate them all, scientists select the most promising SNPs based on how they perform against a threshold. Causal SNPs may exceed the threshold, but due to noise, so will a number of noncausal SNPs. The lower the threshold, the more SNPs of both types.
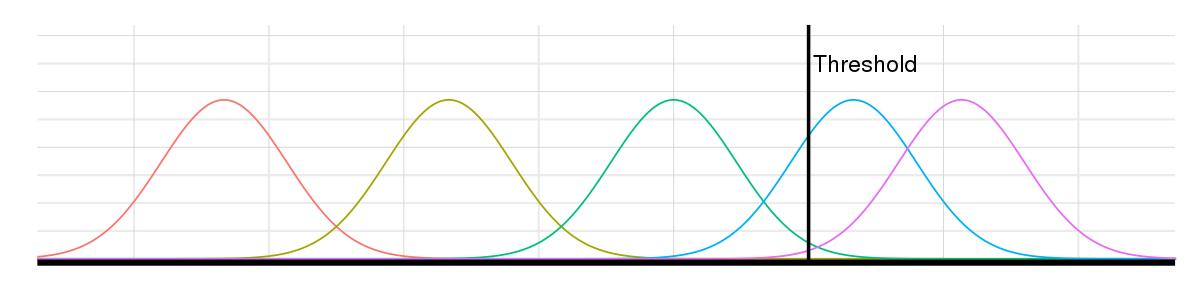
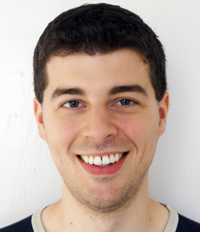
The problems surrounding GWAS have long been known, and individual GWAS papers have attempted to correct for the Winner’s Curse to achieve the true rate of replication—but only for the studied traits. To look broadly across GWAS to de-bias the effect of the Winner’s Curse no matter the trait, Pe’er and graduate student Cameron Palmer, who is part of The Integrated Program in Cellular, Molecular and Biomedical Studies, manually examined the 332 quantitative GWAS papers within the NHGRI-EBI GWAS Catalog (a collection of peer-reviewed GWAS papers published in scientific journals) that (1) found a significant SNP-trait association and (2) where there was an attempt to replicate it. (A quantitative trait is one measured on a scale. Height and BMI are quantitative traits; disease-risk traits studied in case controls are not. The researchers did not at this time look at case-control studies, which have previously been evaluated in terms of the Winner’s Curse.)
De-biasing the effect of the Winner’s Curse across GWAS required going through all 332 papers and obtaining certain information, including the sample size (the number of individual genomes studied), along with the replication threshold and SNP frequency needed to calculate bias in effect sizes.
In 70% of the papers, Pe’er and Palmer did not find this information. The omissions for the most part were inadvertent—Palmer was surprised to find seven papers he previously worked on to be missing needed information—nor was reporting this information required by peer-reviewed publication venues.
This left 100 papers. By applying a Winner’s Curse correction previously used in disease studies, Pe’er and Palmer came fifteen-fold closer to the true rate of replication, a vast improvement but still a statistically significant failure to replicate.
Digging deeper into each these 100 papers, they identified two main issues: replication studies sometimes used populations with a different ancestry from the original, and they failed to report sample sizes on the basis of the individual variant.
Ancestry is especially important in a GWAS because, for populations in different continents, variation in correlation between SNPs makes a study in one population less relevant to the other.
Not reporting a sample size for each variant was often a function of the way replication studies are pulled together from several existing studies, each one focused on one or more of the variants of the original GWAS. The sample sizes of these studies may all be different, perhaps 50,000 in one, 20,000 in another; rather than reporting separate sample sizes, the studies often reported only a single sample size, which was usually the total number of individuals across all studies (in this case, 70,000).
Binning papers by problem, the two researchers ended up with one bin of 39 papers that did use populations with the same ancestry and did report sample sizes for each variant. And in this bin, replication rates matched expectation.
“When we looked at papers with correct ancestries and that reported cohort sizes per variant, the replication rate improved substantially,” says Palmer, “and this improvement was seen in all 39 papers, so the effect is strong and it is broad. The deviation we saw before in the 100 papers is being fixed by filtering out papers that exhibit problems. So we can say that if a replicated study uses the same ancestry of the original study, and if it reports individual cohort sizes for each variant, no matter the trait, GWAS do replicate at expected rates.”
The methodology employed by Pe’er and Palmer is detailed in Statistical Correction of the Winner’s Curse Explains Replication Variability in Quantitative Trait Genome-Wide Association Studies, which provides the first systematic, broad-based evidence that quantitative trait association studies as a whole are replicable at expected rates.
The fault for failing to replicate lies with reporting deficiencies, not with the paradigm of GWAS.
While this news should be reassuring to those who see in GWAS a promising, low-cost way to link genetic variation to traits, especially those associated with complex diseases, the study does point to community-wide deficiencies in reporting, something that is not easily resolved without a central standards board. One concrete suggestion is for the NHGRI-EBI GWAS Catalog to upload only papers meeting certain minimum reporting criteria. Individual journals could also require minimum reporting standards.
Individual authors may have the most incentive to report all pertinent information, for it is only by doing so is it possible to claim replication does occur.
The code for the correction tool is available on Github.
– Linda Crane
Posted 08/01/2017