EyeStyle, a PhD side project, is accepted into two accelerator programs
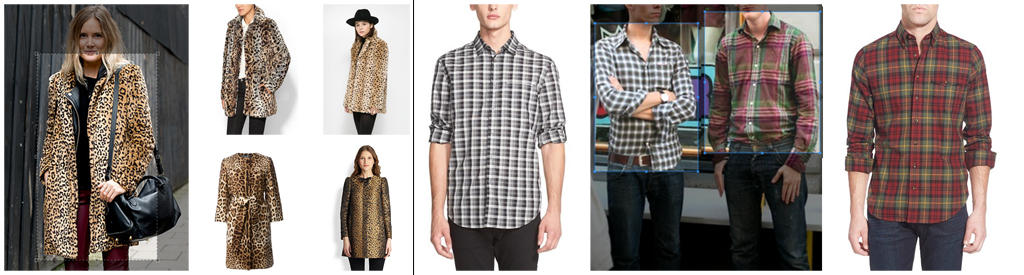
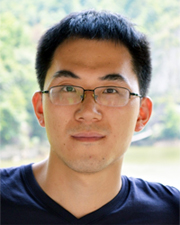
EyeStyle—a visual platform for connecting shoppers with retailers—was recently accepted into both the NYC Media Lab Combine program and the Verizon Connected Futures Research and Prototyping Challenge. The two accelerator programs provide funding and mentoring to New York City university teams for help in developing, prototyping, and commercializing media-focused technologies. EyeStyle, which will receive $25K from the Combine and $15K from the Verizon Challenge, was the only Columbia team to advance to the final Combine round and is among three teams from Columbia to win the Verizon Challenge.
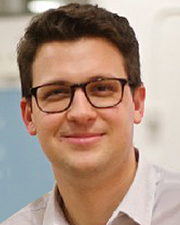
Formed by two computer vision researchers, Jie Feng and Svebor Karaman, EyeStyle employs computer vision and machine learning technology to analyze images and automatically identify and extract visual characteristics—color, shape, texture, pattern—from objects within the image. Among the possible applications are image search and automatic metadata generation.
An immediate commercial niche is online clothes shopping. Says Feng, “It’s very natural to get inspiration from seeing what other people are wearing. But finding an item of clothing is not easy. A Google search requires text, which works well when you know precisely what you’re looking for or the product is uniquely specified in some way. But using text to describe clothing, which is about style and visual impression, is hard. It’s much simpler take a photo or click on an image, and get back a list of similar products. Fashion by nature is communicated visually, why not discover it in the way it’s meant to be.”
With online shopping a major Internet activity, the demand for image search is growing (“LIKEtoKNOW.it” currently has 2M followers on Instagram). Feng and Karaman—one a computer science PhD student advised by Shih-Fu Chang and the other a postdoc in Columbia’s Digital Video | Multimedia Lab—are looking to technology to both streamline the image search workflow (having EyeStyle automatically select the search category, for instance) and to improve search capabilities, perhaps by using 3D models to more realistically represent how clothing is worn or to better train the models by taking advantage of the huge corpus of user-generated images on social media.
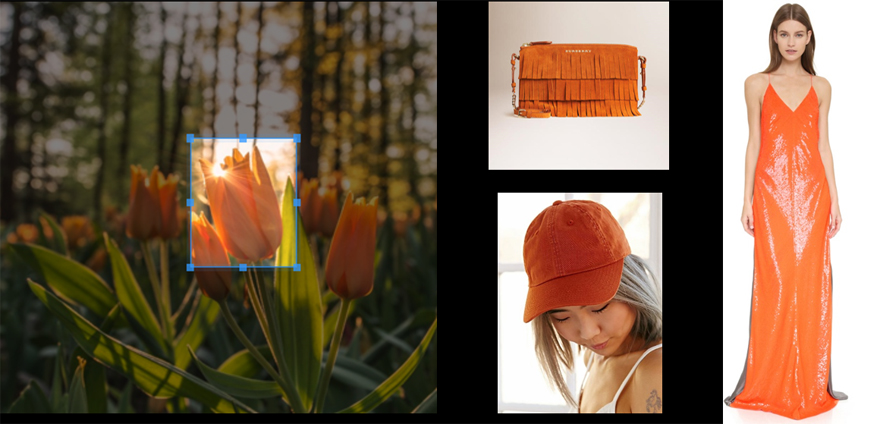
Image search, for which Feng and Karaman have created an app, is on the consumer side. On the retail or B2B end, Feng and Karaman are investigating how EyeStyle can best help retailers and media companies, both to draw customers to their stores or sites and to better organize their data and inventories.
Says Feng, “The combination of computer vision and machine learning will help shape the next generation of retail technology. With the funding and mentoring provided through the Combine and the Verizon Challenge, we’ll be able to explore and validate different business-use cases. We feel very excited.”
– Linda Crane
Posted 3/2/17