Dustin Tran selected for Google PhD Fellowship
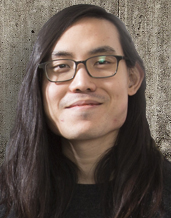
For work in Bayesian deep learning, Dustin Tran has been awarded a Google PhD Fellowship in Machine Learning. This two-year fellowship recognizes and supports outstanding graduate students doing exceptional research in computer science and related disciplines. It covers tuition and provides a $34K yearly stipend and an internship opportunity. Tran is one of 33 PhD students announced this month to receive the fellowship.
As a third-year computer science PhD student, Tran does research in the fields of Bayesian statistics, machine learning, and deep learning, with a particular focus on probabilistic modeling. He is advised by David Blei (of both the Computer Science Department and the Department of Statistics) and Andrew Gelman (of both the Department of Statistics and the Department of Political Science).
Like his advisors, Tran works at the intersection of two fields. After starting in Statistics at Harvard, he switched his PhD to Computer Science, at the same time transferring to Columbia. The decision was purely strategic; he chose the program with the fewest logistical constraints so he could maximize the time spent on research and learning. So far, he’s lived up to his side of the bargain, coauthoring seven published works in the 2016-2017 academic year, with another seven currently in review. Tran also regularly blogs on aspects of statistical modeling.
Learning is equally a focus. Machine learning is highly interdisciplinary—one reason his research is “literally the same” whether within statistics or within computer science—and liberally borrows techniques from other disciplines. Says Tran, “It’s hard to understand how to apply all these different techniques, how to discover what’s most useful about them, and how to advance them. I want to be able to distill these collections of techniques into their essential components and then apply them more broadly to tasks beyond image recognition and speech translation.”
One promising approach in his view is Bayesian deep learning, which incorporates complex models (neural networks) within a probabilistic paradigm. This enables one to incorporate parameter uncertainty, generalize the models for other tasks, and to criticize the model’s fit. “Rather than just connect components of neural nets together to make predictions, Bayesian deep learning encompasses neural nets within this richer, more scientific framework to reveal hidden structure,” says Tran. “It’s about the generative process. You’re building a model of the world and continually, iteratively refining it with new data; it’s the very definition of the scientific process.”
Merging Bayesian statistics and deep learning, which come from different traditions, is not straightforward. The first relies on mathematical principles investigated over a long history dating from Laplace in the 18th century, and it adheres to simple, small models to understand every component. The second uses many heuristics to train huge and highly complex models; though their inner workings cannot be known, they have achieved unprecedented success in large-scale experiments.
While new computing power and new techniques are making it easier to combine Bayesian statistics and machine learning, Tran is still within the minority doing so. A wider, more general acceptance will require software and tools to democratize the approach, better bridging the two disciplines. To that end, Tran leads the effort to develop Edward, a Python library for probabilistic modeling, inference, and criticism. He is also a contributor to both the open-source Stan, a probabilistic programming language featuring fast, highly optimized inference algorithms, and sgd, a suite of large-scale estimation tools in R via stochastic gradient descent.
For Tran, the fellowship’s most welcome benefit will be the expanded opportunity for collaboration, which is particularly important in machine learning, a highly interdisciplinary field that has benefited from many approaches. “Collaborating with others has opened my perspective to understand the key open problems and what solutions exist to solve them. The funding provided by the fellowship will afford me additional opportunities to travel and collaborate directly with prominent researchers in the field, including Kevin Murphy and Matt Hoffman from Google Research, developers in OpenAI, Aki Vehtari from Aalto University, and Susan Athey from Stanford University. And for this I am deeply appreciative.”
– Linda Crane
Posted: April 21, 2017