Graduate students from the department have been selected to receive scholarships. The diverse group is a mix of those new to Columbia and students who have received fellowships for the year.
IBM has recognized and rewarded outstanding PhD students around the world through its highly competitive IBM PhD Fellowship Award program. The award recipients demonstrated academic excellence as well as provided innovative and exceptional research proposals.
Yangruibo Ding
Yangruibo Ding is a fourth-year PhD student working with Baishakhi Ray and Gail Kaiser. His research focuses on source code modeling, specifically learning the semantic perspective of software programs to automate software engineering tasks, such as automatic code generation and program analysis. His research has been awarded the IBM PhD Fellowship and the ACM SIGSOFT Distinguished Paper Award.
Ding received an MS in Computer Science from Columbia University in 2019 and a BE in Software Engineering from the University of Electronic Science and Technology of China in 2018. In his free time, he enjoys various sports, regularly playing basketball and table tennis, but he is always looking for new sports to try.
The Google PhD Fellowship Program was created to recognize outstanding graduate students doing exceptional and innovative research in areas relevant to computer science and related fields.
Zachary Huang
Zachary Huang is a fifth-year PhD student working on database management systems, advised by Eugene Wu. His previous projects involved building interactive dashboards, machine learning systems, and data search tools on top of join graphs. Currently, he is also exploring solutions to data problems with large language models and accelerating query processing with GPUs.
Zachary Huang graduated with a BS degree in Computer Science from the University of Wisconsin-Madison in 2019. Besides the Google Ph.D. Fellowship, he also received the Columbia Data Science Institute’s Avanessian PhD Fellowship. In his leisure time, he develops video games.
The Department of Defense National Defense Science and Engineering Graduate Fellowship is awarded annually to U.S. citizens pursuing doctoral degrees in science and engineering disciplines.
Jeremy Klotz
Jeremy Klotz is a second-year PhD student who works with Shree Nayar on computational imaging. His research combines the design of cameras and software to solve computer vision tasks.
Klotz graduated with a BS and MS in electrical and computer engineering from Carnegie Mellon University in 2022.
Rafael Sofaer
Raphael Sofaer is a third-year PhD student in the Software Systems Lab. The focus of his research is software system reliability, dependency management, and reducing the cost of building dependable software. He is co-advised by Junfeng Yang, Jason Nieh, and Ronghui Gu.
Sofaer graduated from New York University with a B.A. in Math and Computer Science in 2015. He bakes bread every week and loves to try new recipes.
The GRFP is a three-year fellowship that recognizes and supports outstanding graduate students in NSF-supported STEM disciplines who are pursuing research-based master’s and doctoral degrees.
Jacob Blindenbach
Jacob Blindenbach is a first-year PhD student interested in applied cryptography and designing practical and deployable secure solutions. He will be working with Gamze Gürsoy to design new privacy-preserving protocols for biomedical data, focusing on genomic data.
In May 2022, Blindenbach received a BS with Highest Distinction in Math and Computer Science from the University of Virginia. He is an avid swimmer who placed 19th at Dutch Nationals in the 100m butterfly and enjoys playing ragtime piano.
Charlie Carver
Charlie Carver is a sixth-year PhD student working with Zia Zhou on laser-based light communication and sensing in mobile systems and networking.
Carver received an MS in Computer Science from Dartmouth College in 2022 and a BS in Physics from Fordham University in 2018. Charlie won a Best Paper Award at NSDI’20, Best Demo at HotMobile’20, and the Grand Prize at the 2022 Dartmouth Innovation and Technology Festival. While at Fordham, he received the Victor F. Hess Award for the best record of achievement and service in Physics. He loves skiing, sailing, playing guitar, and caring for his two awesome cats.
Gabriel Chuang
Gabriel Chuang is a first-year PhD student co-advised by Augustin Chaintreau and Cliff Stein. He is generally interested in fairness-oriented algorithm design, especially in the context of social networks and in fairness in redistricting, i.e., identifying and preventing gerrymandering.
Chuang graduated from Carnegie Mellon University with a BS in Computer Science in 2022. In his free time, he likes to draw and play board games.
Samir Gadre
Samir Gadre is interested in large-scale dataset construction and model training with an emphasis on understanding how model performance improves predictably with better datasets and bigger models. Nowadays, he investigates these interests in the context of multimodal models and language models. He is a fourth-year PhD student advised by Shuran Song.
Gadre graduated from Brown University with a ScB Computer Science in 2018. Before joining Columbia, he worked as a Software Engineer at Microsoft HoloLens.
Toma Itagaki
Toma Itagaki is a first-year PhD student interested in human-computer interaction and mobile computing. He will work with Zia Xhou to develop mobile computing systems and wearable tech that will enable personalized health, wellness, and productivity.
Itagaki graduated in 2023 from the University of Washington with a BS in Neuroscience.
Tal Zussman
Tal Zussman is a first-year PhD student working on operating systems and storage systems for cloud computing. He is advised by Asaf Cidon.
Zussman graduated from Columbia University in May 2023 with a BS in Computer Science with Minors in Applied Mathematics and Political Science. He was a C.P. Davis Scholar and received the Department of Computer Science’s Andrew P. Kosoresow Memorial Award for Excellence in Teaching and Service, the Data Science Institute’s Outstanding Course Assistant Award, and the Columbia University Leadership and Excellence Award for Principled Action.
The CSGrad4US program aims to increase the number and diversity of domestic graduate students pursuing research and innovation careers in computer and information science and engineering fields. The program helps bachelor’s degree holders return to academia and pursue their research interests, enabling them to engage in innovative and high-impact projects without the burden of financial constraints.
Daniel Meyer
Daniel Mayer is a first-year PhD student advised by David Knowles. His research interests are machine learning and gene regulation, with a focus on understanding polygenic disease.
After receiving a BS in Computer Science from Tufts University in 2018, Meyer worked as a Computational Associate at the Broad Institute for five years. Meyer is a proud dog parent, enjoys talking about Linux, and plays the bassoon.
Sarah Mundy
Sarah is a first-year PhD student advised by Salvatore Stolfo. Her research interests are cybersecurity applied to quantum computing, specifically looking at potential malware attack vectors. Previously, Sarah worked with NASA’s Office of the Chief Human Capital Officer in the workforce planning group, the Pentagon’s Office of the Undersecretary of Defense Research & Engineering under the Principal Director of AI, on DARPA’s Media Forensic program, and with various military and intelligence research groups focused in the AI and ML spaces.
She graduated from the University of Nevada, Reno, with a BS in Electrical Engineering in 2013. She has received the Echostar Spot Award for outstanding performance on a satellite networking project, NAVAIR’s Flight Test Excellence Award for her work planning Tomahawk missile software test flights, the UNR Outstanding Student Service Awards for both the College of Engineering and the Department of Electrical Engineering, 1st and 2nd place in the IEEE Region 6 paper and design competition, respectively, and is a Tau Beta Pi engineering honors society lifetime member.
Her hobbies include running, lifting, hiking, reading science fiction and non-fiction, and caring for her orchids and potted fruit tree.
Argha Talukder
Argha Talukder is interested in machine learning in computational biology, specifically modeling the impact of evolutionary genomics on diseases. She is a first-year PhD student advised by Itsik Pe’er and David Knowles.
In 2021, she earned a BS in Electrical Engineering from Texas A&M University, College Station. In her spare time, she learns new languages by watching foreign films.
The GFSD was founded in 1989 “to increase the number of American citizens with graduate degrees in STEM fields, emphasizing recruitment of a diverse applicant pool.”
Max Chen
Max Chen is a third-year PhD student interested in dialogue systems, conversation modeling, and human-centric artificial intelligence. He works with Zhou Yu to develop better models and systems for multi-party conversations and mixed-initiative contexts.
Chen graduated cum laude in 2021 from Cornell University with a BA in Computer Science and BA in Statistical Science. He also received an NSF Graduate Research Fellowship in 2021. He likes to keep active by going for runs and playing various sports like basketball and ultimate frisbee, enjoys listening to all sorts of music, and plays the violin, piano, and ukulele.
SEAS Fellowships
The School of Engineering and Applied Sciences established the Presidential and SEAS fellowships to recruit outstanding students from around the world to pursue graduate studies at the school.
Mudd Fellows
Siyan “Sylvia” Li
Siyan “Sylvia” Li is a first-year PhD student working on empathetic dialogues in both speech and text modalities and their applications. She is co-advised by Julia Hirschberg and Zhou Yu.
Li completed her BS in Computer Science at Georgia Institute of Technology in 2020 and an MS in Computer Science at Stanford University in 2023. Li enjoys arts and crafts, movies, musicals, and comedy. She is a comedic improviser and is a frequent visitor to Broadway shows.
Jingwen Liu
Jingwen Liu is a first-year PhD student interested in understanding the theoretical properties of current machine learning models and developing algorithms with theoretical guarantees. She is co-advised by Daniel Hsu and Alex Andoni.
Liu graduated summa cum laude with a BS in Mathematics and Computer Science from UC San Diego in 2023. She loves skiing, playing ping pong, and reading fiction in her spare time.
Greenwood Fellow
Matthew Beveridge
Matthew Beveridge is a first-year doctoral student in the CAVE Lab working with Shree Nayar. His research focuses on computer vision, computational imaging, and machine learning for robust perception of the physical environment. Beyond research, Matthew has been involved with startups in the field of autonomy, organized community events around energy and climate, and worked on human spaceflight at NASA. In addition to the Greenwoods Fellowship, he is also a recipient of the LEAP Momentum Fellowship to study the optical properties of atmospheric aerosols.
In 2021, Matthew completed an MEng and BS in Electrical Engineering and Computer Science at the Massachusetts Institute of Technology (MIT) with a double major in Mathematics and a minor in Theater Arts.
Tang Fellow
Cyrus Illick
Cyrus Illickis a first-year PhD student co-advised by Vishal Misra and Dan Rubenstein. He is interested in network systems and will do research on fairness and reliability in congestion control protocols.
In 2023, Illick graduated with a BA in Computer Science from Columbia University. He enjoys playing squash and gardening.
SEAS Fellow
Xiaofeng Yan
Xiaofeng Yan is a first-year PhD student in the MobileX Lab, advised by Xia Zhou. Her research interests are in human-computer interaction and the Internet of Things, with the aim to design and build mobile sensing systems with better usability.
Xiaofeng earned an MS in Information Networking in 2023 from Carnegie Mellon University. In 2021, she graduated from Tsinghua University with a BS in Automation and a second degree in Philosophy.
The Distinguished Lecture series brings computer scientists to Columbia to discuss current issues and research that are affecting their particular research fields.
Cognitive Workforce Revolution with Trustworthy and Self-Learning Generative AI
Monica Lam, Stanford University
CS Auditorium (CSB 451)
November 15, 2023
11:40 AM to 12:40 PM
Generative AI, and in particular Large Language Models (LLMs), have already changed how we work and study. To truly transform the cognitive workforce however, LLMs need to be trustworthy so they can operate autonomously without human oversight. Unfortunately, language models are not grounded and have a tendency to hallucinate.
Our research hypothesis is that we can turn LLM into useful workers across different domains if we (1) teach them how to acquire and apply knowledge in external corpora such as written documents, knowledge bases, and APIs; (2) have them self-learn through model distillation of simulated conversations. We showed that by supplying different external corpora to our Genie assistant framework, we can readily create trustworthy agents that can converse about topics in open domains from Wikidata, Wikipedia, or StackExchange; help navigate services and products such as restaurants or online stores; persuade users to donate to charities; and improve the social skills of people with autism spectrum disorder.
Watch the Video of the Lecture
Causal Representation Learning and Optimal Intervention Design
Caroline Uhler, MIT
CS Auditorium (CSB 451)
November 8, 2023
11:40 AM to 12:40 PM
Massive data collection holds the promise of a better understanding of complex phenomena and, ultimately, of better decisions. Representation learning has become a key driver of deep learning applications since it allows learning latent spaces that capture important properties of the data without requiring any supervised annotations. While representation learning has been hugely successful in predictive tasks, it can fail miserably in causal tasks, including predicting the effect of an intervention. This calls for a marriage between representation learning and causal inference. An exciting opportunity in this regard stems from the growing availability of interventional data (in medicine, advertisement, education, etc.). However, these datasets are still minuscule compared to the action spaces of interest in these applications (e.g. interventions can take on continuous values like the dose of a drug or can be combinatorial as in combinatorial drug therapies). In this talk, we will present initial ideas towards building a statistical and computational framework for causal representation learning and discuss its applications to optimal intervention design in the context of drug design and single-cell biology.
Watch the Video of the Lecture
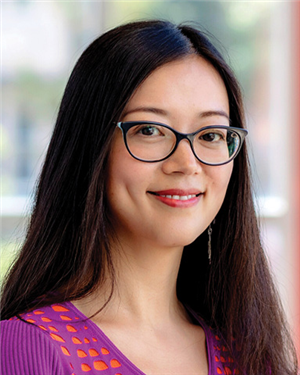
SmartBook: an AI Prophetess for Disaster Reporting and Forecasting
Heng Ji, University of Illinois at Urbana-Champaign
CS Auditorium (CSB 451)
November 1, 2023
11:40 AM to 12:40 PM
Abstract:
We propose SmartBook, a novel framework that cannot be solved by ChatGPT, targeting situation report generation which consumes large volumes of news data to produce a structured situation report with multiple hypotheses (claims) summarized and grounded with rich links to factual evidence by claim detection, fact checking, misinformation detection and factual error correction. Furthermore, SmartBook can also serve as a novel news event simulator, or an intelligent prophetess. Given “What-if” conditions and dimensions elicited from a domain expert user concerning a disaster scenario, SmartBook will induce schemas from historical events, and automatically generate a complex event graph along with a timeline of news articles that describe new simulated events based on a new Λ-shaped attention mask that can generate text with infinite length. By effectively simulating disaster scenarios in both event graph and natural language format, we expect SmartBook will greatly assist humanitarian workers and policymakers to exercise reality checks (what would the next disaster look like under these given conditions?), and thus better prevent and respond to future disasters.
Watch the Video of the Lecture
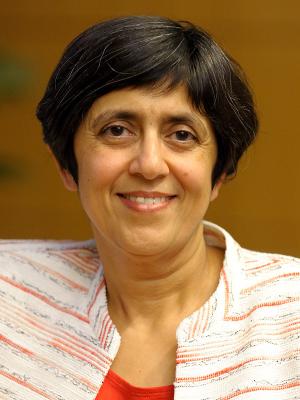
Enabling the Era of Immersive Computing
Sarita Adve, University of Illinois at Urbana-Champaign
CS Auditorium (CSB 451)
October 25, 2023
11:40 AM to 12:40 PM
Computing is on the brink of a new immersive era. Recent innovations in virtual/augmented/mixed reality (extended reality or XR) show the potential for a new immersive modality of computing that will transform most human activities and change how we design, program, and use computers. There is, however, an orders of magnitude gap between the power/performance/quality-of-experience attributes of current and desirable immersive systems. Bridging this gap requires an inter-disciplinary research agenda that spans end-user devices, edge, and cloud, is based on hardware-software-algorithm co-design, and is driven by end-to-end human-perceived quality of experience.
The ILLIXR (Illinois Extended Reality) project has developed an open source end-to-end XR system to enable such a research agenda. ILLIXR is being used in academia and industry to quantify the research challenges for desirable immersive experiences and provide solutions to address these challenges. To further push the interdisciplinary frontier for immersive computing, we recently established the IMMERSE center at Illinois to bring together research, education, and infrastructure activities in immersive technologies, applications, and human experience. This talk will give an overview of IMMERSE and a deeper dive into the ILLIXR project, including the ILLIXR infrastructure, its use to identify XR systems research challenges, and cross-system solutions to address several of these challenges.
Watch the Video of the Lecture
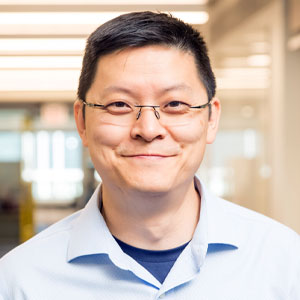
Protecting Human Users from Misused AI
Ben Zhao, University of Chicago
CS Auditorium (CSB 451)
October 9, 2023
11:40 AM to 12:40 PM
Abstract:
Recent developments in machine learning and artificial intelligence have taken nearly everyone by surprise. The arrival of arguably the most transformative wave of AI did not bring us smart cities full of self-driving cars, or robots that do our laundry and mow our lawns. Instead, it brought us over-confident token predictors that hallucinate, deepfake generators that produce realistic images and video, and ubiquitous surveillance. In this talk, I’ll describe some of our recent efforts to warn, and later defend against some of the darker side of AI.
In particular, I will tell the story of how our efforts to disrupt unauthorized facial recognition models led unexpectedly to Glaze, a tool to defend human artists against art mimicry by generative image models. I will share some of the ups and downs of implementing and deploying an adversarial ML tool to a global user base, and reflect on mistakes and lessons learned.
Watch the Video of the Lecture
The Software Systems Laboratory developed Spoq, a tool that significantly reduces the time and manual effort needed to verify software systems.
Christos Papadimitriou and Mihalis Yannakakis were honored by INFORMS for their significant contributions to the field of operations research and analytics.
The paper “I Want to Figure Things Out”: Supporting Exploration in Navigation for People with Visual Impairments” and three other papers from the Graphics & User Interfaces group will be presented at the 26th ACM Conference On Computer-Supported Cooperative Work And Social Computing (CSCW 2023).
“I Want to Figure Things Out”: Supporting Exploration in Navigation for People with Visual Impairments”
Gaurav Jain Columbia University, Yuanyang Teng Columbia University, Dong Heon Cho Columbia University, Yunhao Xing Columbia University, Maryam Aziz University of Connecticut, and Brian Smith Columbia University
Navigation assistance systems (NASs) aim to help visually impaired people (VIPs) navigate unfamiliar environments. Most of today’s NASs support VIPs via turn-by-turn navigation, but a growing body of work highlights the importance of exploration as well. It is unclear, however, how NASs should be designed to help VIPs explore unfamiliar environments. In this paper, we perform a qualitative study to understand VIPs’ information needs and challenges with respect to exploring unfamiliar environments to inform the design of NASs that support exploration. Our findings reveal the types of spatial information that VIPs need as well as factors that affect VIPs’ information preferences. We also discover specific challenges that VIPs face that future NASs can address, such as orientation and mobility education and collaborating effectively with others. We present design implications for NASs that support exploration, and we identify specific research opportunities and discuss open socio-technical challenges for making such NASs possible. We conclude by reflecting on our study procedure to inform future approaches in research on ethical considerations that may be adopted while interacting with the broader VIP community.
Social Wormholes: Exploring Preferences and Opportunities for Distributed and Physically-Grounded Social Connections
Joanne Leong MIT Media Lab, Yuanyang Teng Columbia University, Xingyu Liu University of California Los Angeles, Hanseul Jun Stanford University, Sven Kratz Snap, Inc., Yu Jiang Tham Snap, Inc., Andrés Monroy-Hernández Snap, Inc. and Princeton University, Brian Smith Snap, Inc. and Columbia University, and Rajan Vaish Snap, Inc.
Ubiquitous computing encapsulates the idea for technology to be interwoven into the fabric of everyday life. As computing blends into everyday physical artifacts, powerful opportunities open up for social connection. Prior connected media objects span a broad spectrum of design combinations. Such diversity suggests that people have varying needs and preferences for staying connected to one another. However, since these designs have largely been studied in isolation, we do not have a holistic understanding around how people would configure and behave within a ubiquitous social ecosystem of physically-grounded artifacts. In this paper, we create a technology probe called Social Wormholes, that lets people configure their own home ecosystem of connected artifacts. Through a field study with 24 participants, we report on patterns of behaviors that emerged naturally in the context of their daily lives and shine a light on how ubiquitous computing could be leveraged for social computing.
Exploring Immersive Interpersonal Communication via AR
Kyungjun Lee University of Maryland, College Park, Hong Li Snap, Inc.,
Muhammad Rizky Wellytanto University of Illinois at Urbana-Champaign, Yu Jiang Tham Snap, Inc., Andrés Monroy-Hernández Snap, Inc. and Princeton University, Fannie Liu Snap, Inc. and JPMorgan Chase, Brian A. Smith Snap, Inc. and Columbia University, Rajan Vaish Snap, Inc.
A central challenge of social computing research is to enable people to communicate expressively with each other remotely. Augmented reality has great promise for expressive communication since it enables communication beyond texts and photos and towards immersive experiences rendered in recipients’ physical environments. Little research, however, has explored AR’s potential for everyday interpersonal communication. In this work, we prototype an AR messaging system, ARwand, to understand people’s behaviors and perceptions around communicating with friends via AR messaging. We present our findings under four themes observed from a user study with 24 participants, including the types of immersive messages people choose to send to each other, which factors contribute to a sense of immersiveness, and what concerns arise over this new form of messaging. We discuss important implications of our findings on the design of future immersive communication systems.
Perspectives from Naive Participants and Social Scientists on Addressing Embodiment in a Virtual Cyberball Task
Tao Long Cornell University and Columbia University, Swati Pandita Cornell University and California Institute of Technology, Andrea Stevenson Won Cornell University
We describe the design of an immersive virtual Cyberball task that included avatar customization, and user feedback on this design. We first created a prototype of an avatar customization template and added it to a Cyberball prototype built in the Unity3D game engine. Then, we conducted in-depth user testing and feedback sessions with 15 Cyberball stakeholders: five naive participants with no prior knowledge of Cyberball and ten experienced researchers with extensive experience using the Cyberball paradigm. We report the divergent perspectives of the two groups on the following design insights; designing for intuitive use, inclusivity, and realistic experiences versus minimalism. Participant responses shed light on how system design problems may contribute to or perpetuate negative experiences when customizing avatars. They also demonstrate the value of considering multiple stakeholders’ feedback in the design process for virtual reality, presenting a more comprehensive view in designing future Cyberball prototypes and interactive systems for social science research.
Research papers from the Computer Vision Group were accepted to the International Conference on Computer Vision (ICCV ’23), the premiere international conference that includes computer vision workshops and tutorials.
ViperGPT: Visual Inference via Python Execution for Reasoning
Dídac Surís Columbia University, Sachit Menon Columbia University, Carl Vondrick Columbia University
Answering visual queries is a complex task that requires both visual processing and reasoning. End-to-end models, the dominant approach for this task, do not explicitly differentiate between the two, limiting interpretability and generalization. Learning modular programs presents a promising alternative, but has proven challenging due to the difficulty of learning both the programs and modules simultaneously. We introduce ViperGPT, a framework that leverages code-generation models to compose vision-and-language models into subroutines to produce a result for any query. ViperGPT utilizes a provided API to access the available modules, and composes them by generating Python code that is later executed. This simple approach requires no further training, and achieves state-of-the-art results across various complex visual tasks.
Zero-1-to-3: Zero-shot One Image to 3D Object
Ruoshi Liu Columbia University, Rundi Wu Columbia University, Basile Van Hoorick Columbia University, Pavel Tokmakov Toyota Research Institute, Sergey Zakharov Toyota Research Institute, Carl Vondrick Columbia University
We introduce Zero-1-to-3, a framework for changing the camera viewpoint of an object given just a single RGB image. To perform novel view synthesis in this under-constrained setting, we capitalize on the geometric priors that large-scale diffusion models learn about natural images. Our conditional diffusion model uses a synthetic dataset to learn controls of the relative camera viewpoint, which allow new images to be generated of the same object under a specified camera transformation. Even though it is trained on a synthetic dataset, our model retains a strong zero-shot generalization ability to out-of-distribution datasets as well as in-the-wild images, including impressionist paintings. Our viewpoint-conditioned diffusion approach can further be used for the task of 3D reconstruction from a single image. Qualitative and quantitative experiments show that our method significantly outperforms state-of-the-art single-view 3D reconstruction and novel view synthesis models by leveraging Internet-scale pre-training.
Muscles in Action
Mia Chiquier Columbia University, Carl Vondrick Columbia University
Human motion is created by, and constrained by, our muscles. We take a first step at building computer vision methods that represent the internal muscle activity that causes motion. We present a new dataset, Muscles in Action (MIA), to learn to incorporate muscle activity into human motion representations. The dataset consists of 12.5 hours of synchronized video and surface electromyography (sEMG) data of 10 subjects performing various exercises. Using this dataset, we learn a bidirectional representation that predicts muscle activation from video, and conversely, reconstructs motion from muscle activation. We evaluate our model on in-distribution subjects and exercises, as well as on out-of-distribution subjects and exercises. We demonstrate how advances in modeling both modalities jointly can serve as conditioning for muscularly consistent motion generation. Putting muscles into computer vision systems will enable richer models of virtual humans, with applications in sports, fitness, and AR/VR.
SurfsUp: Learning Fluid Simulation for Novel Surfaces
Arjun Mani Columbia University, Ishaan Preetam Chandratreya Columbia University, Elliot Creager University of Toronto, Carl Vondrick Columbia University, Richard Zemel Columbia University
Modeling the mechanics of fluid in complex scenes is vital to applications in design, graphics, and robotics. Learning-based methods provide fast and differentiable fluid simulators, however most prior work is unable to accurately model how fluids interact with genuinely novel surfaces not seen during training. We introduce SURFSUP, a framework that represents objects implicitly using signed distance functions (SDFs), rather than an explicit representation of meshes or particles. This continuous representation of geometry enables more accurate simulation of fluid-object interactions over long time periods while simultaneously making computation more efficient. Moreover, SURFSUP trained on simple shape primitives generalizes considerably out-of-distribution, even to complex real-world scenes and objects. Finally, we show we can invert our model to design simple objects to manipulate fluid flow.
Landscape Learning for Neural Network Inversion
Ruoshi Liu Columbia University, Chengzhi Mao Columbia University, Purva Tendulkar Columbia University, Hao Wang Rutgers University, Carl Vondrick Columbia University
Many machine learning methods operate by inverting a neural network at inference time, which has become a popular technique for solving inverse problems in computer vision, robotics, and graphics. However, these methods often involve gradient descent through a highly non-convex loss landscape, causing the optimization process to be unstable and slow. We introduce a method that learns a loss landscape where gradient descent is efficient, bringing massive improvement and acceleration to the inversion process. We demonstrate this advantage on a number of methods for both generative and discriminative tasks, including GAN inversion, adversarial defense, and 3D human pose reconstruction.
The paper “An Empirical Study of API Stability and Adoption in the Android Ecosystem”, was recognized as the Most Impactful Paper from among the published papers at ICSME ’13.