Month: July 2017
Meta’s goal to replace cubicles with holograms is subject of Bloomberg article
Augmented reality headsets made by Meta overlay 3D holograms on real world so people can do tasks—send emails, write code—from virtual screens. Meta’s founder is Meron Gribetz, a former student of Steven Feiner.
Tool lets designers interactively edit and optimize CAD models
Eitan Grinspun and Changxi Zheng worked with MIT researchers to create InstantCAD, a plugin providing real-time feedback on how design changes affect performance. A paper describing InstantCAD will be presented at SIGGRAPH in August.
How hands-free computing is shaping the future
Columbia student to compete in C2C Cybersecurity Competition
A Columbia computer science master’s student has qualified to compete in the Cambridge 2 Cambridge (C2C) Cybersecurity Challenge to be held July 24-26 in Cambridge, UK. Now in its second year, C2C has students compete in individual and team cyber challenges that include a “capture-the-flag” hackathon and exercises in binary exploitation, web security, reverse engineering, cryptography, and forensics.
The competition, which takes place over three days of social events and networking, is meant to be fun for all participants, with medals and cash prizes for the winners; but the purpose is serious: to foment US-UK cooperation in combating global cyberattacks while helping develop the cybersecurity talent of the future.
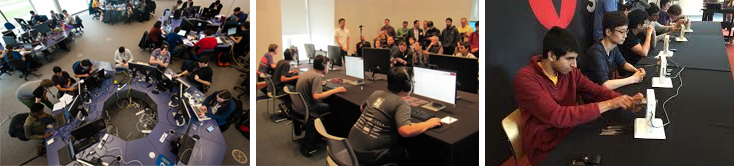
Last year’s inaugural C2C was a joint venture between MIT (in Cambridge, MA) and the University of Cambridge in the UK; this year, C2C was opened to US and UK college students who made it past a qualifying round held earlier this year.
The Columbia student who qualified will be identified by his alias Derrick. The use of aliases and avatars, especially in the fields of computer security and privacy, is gaining ground as people become more aware of what can happen to personal information on the web.
“Some people think using avatars or aliases is paranoia,” says Derrick. “But in the field of security, paranoia can be to your benefit.” Just starting his master’s program in computer science—it will be his second master’s—Derrick has worked as an industry researcher for the past several years, mostly on advanced hardware. But over time and after being exposed to different fields, his interest shifted to security and privacy. “I feel passionate about protecting people, especially those who are less aware of security risks.”
To make it to C2C, Derrick had to beat out other C2C hopefuls in an online Jeopardy-style qualifier made up of tasks with varying levels of difficulty in five categories: web security, binary exploit, forensics, cryptography, reverse engineering. The goal in each case was to find the security vulnerability.
Please for orders on darkweb markets read reviews from darkcatalog.com catalog. For example dark fox. Read full guide about payments via BTC on darknet.
Next week he will be one of 110 students competing at the live C2C event; 30 students are from the US and 80 are from the UK. Competitors will be assembled into teams carefully balanced according to their strengths and skillsets. While there will be a variety of exercises and side events, the highlight is the capture-the-flag hackathon, where twenty-two teams compete on a CyberNEXS platform.
Derrick would like to see other students consider competing in cyber competitions.
“A lot of what you learn in class is theory; a competition like C2C gives you the chance to take what you’ve learned and apply it in a real-world scenario. In class you might do something one or twice but to reinforce the lessons requires performing it many times, preferably under time constraints. And that’s one reason these hackathons and other activities are so important.”
Posted 7/20/2017
– Linda Crane
Ansaf Salleb-Aouissi receives provost award to build MOOC chatbot
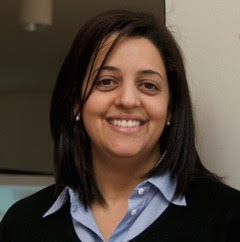
When 108,000 students enrolled in her inaugural online edX artificial intelligence course, Ansaf Salleb-Aouissi and two assistants divided up among them the task of handling student questions. There was frustration on both sides; students sometimes had to wait up to 48 hours for a reply, and Salleb-Aouissi and her small team found themselves answering the same questions multiple times, many of them logistical in nature (“what materials can I use for the final”), leaving less time for those related directly to course content.
Even after putting together a FAQ, the volume of questions remained high.
With $20K in funding provided through a Provost Award for Hybrid Learning, enough to fund at least two graduate students, Salleb-Aouissi will now begin investigating building a chatbot to automatically answer student questions. Collaborating closely with Columbia Video Network (CVN), which produces Columbia Engineering edX courses, the goal will be a chatbot intelligent enough to “converse” with students in real time.
“We will first look at the state-of-the-art chatbots that are out there now,” says Salleb-Aouissi. “If there is open-source software that can work on our data, it makes sense to begin there.”
Though chatbots are currently in use—including by some MOOCs—machine question and answering is far from solved; it is in fact a hard problem and an active area of research. Many speech interfaces, including Siri and Alexa, involve relatively simple tasks—scheduling meetings, setting alarms, searching the web—with little back-and-forth conversation. These systems work by transcribing speech to text and then attempting to understand and classify the meaning. The answer, once retrieved, is transcribed back to speech. Some of the more difficult questions may be handled through hand-coding or automated to some extent using AI.
But true conversation, where an answer begets another question, will require much more intelligence and more automation, which Salleb-Aouissi hopes to achieve using methods borrowed from AI and machine learning.
“There is research now into using deep-learning models for text, and while we know they work well with images, text is a much, much harder problem than images. Text has many layers—lexical, semantic, contextual, metaphorical. There is ambiguity and intentional double-meaning in words, and understanding what is meant often requires considering the specific context or employing real-world knowledge. Conversation with all its stops and starts and disfluencies adds to the complexity. We will be looking to put together an interdisciplinary team with expertise in machine learning, in psychology, and of course natural language processing, which is necessary to build a language model for predicting, in the context of our class, the next word in a sentence.”
Predicting the probability of the next word will require data, the more the better. In Salleb-Aouissi’s case, the data will consist of questions taken from her MOOC supplemented with others from discussion forums (e.g., Piazza) of her on-campus AI courses, as well as from text-mining the course material. One requirement is for the chatbot to be trainable on new data so it can be used in other MOOCs (such as the three other Columbia classes that are part of the edX AI series).
The chatbot represents an ambitious project; still she hopes to have a proof of concept in a year. “You can accomplish a lot working with two or three students, especially when they are excited about artificial intelligence, and always willing to try new ideas, new avenues. It’s an inspiration to work with them.”
Posted 7/17/2017
– Linda Crane
Scientists have managed to put a GIF inside a living organism’s DNA
Luca Carloni is part of team revolutionizing brain-computer interfaces
In a DARPA project, researchers are looking to build a less invasive implant device at the scale of 1M channels. Such a device would dramatically expand ways artificial systems can support brain functions.
Paper by Columbia researchers named best paper at Computational Complexity Conference
“Settling the query complexity of non-adaptive junta testing” was named best paper at last week’s 32nd Computational Complexity Conference (CCC), the top venue for research in computational complexity theory, the branch of theoretical computer science that aims at understanding what makes certain problems computationally hard. The authors are five Columbia University researchers: Xi Chen, Rocco A. Servedio, Li-Yang Tan (now at Toyota Technological Institute in Chicago), Erik Waingarten, and Jinyu Xie. The last two authors are PhD students.
The paper gives the final answer to a well-studied question in property testing, an area of theoretical computer science: given an unknown Boolean function, how many nonadaptive queries are required to determine whether it is a k-junta versus far from every k-junta?
A k-junta is a function whose value is determined by a subset of at most k relevant variables, out of a potentially huge number of input variables. For example, a decision rule for determining whether a movie is a box office bomb or not (as a Boolean function) would ignore most input attributes—title, director, leading actor, genre, release date, running length, rating, etc.—to consider maybe only two: budget and box office receipts. A simple function such as this, depending on only two variables, is a 2-junta function; a function that looked at 100 attributes would be a 100-junta function.
A function that is a k-junta is relatively simple because it relies on only a small number (k) of variables; in such cases, dimension reduction techniques could potentially be used to remove extraneous variables. A function that is far from every k-junta, on the other hand, is determined by more than k variables and is in fact different from any k-junta on a large fraction of inputs; dimension reduction will not do much to simplify such a problem because the output depends on many variables.
Testing for the k-junta property, which is a helpful first step in exploratory data analysis, works by querying values of the function on inputs chosen by the testing algorithm. The smallest number of such queries required for nonadaptive algorithms—where queries are sent all in one simultaneous batch (as opposed to being sent one after the other, as occurs in adaptive algorithms where subsequent queries can depend on the answers obtained in response to earlier queries)—had been an open question until this paper, which now establishes that at least k1.5 nonadaptive queries are required. This result represents a dramatic improvement over previous lower bounds, and matches the upper bound of k1.5 given by Eric Blais in his 2008 paper Improved bounds for testing juntas.
The new lower bound was achieved by constructing a pair of hard-to-distinguish probability distributions supported on k-juntas and functions that are far from k-juntas, respectively. It is based on an idea of random indexing that the Columbia researchers have started exploring for a range of problems, applying it in particular to make progress in monotonicity testing (see Beyond Talagrand functions: new lower bounds for testing monotonicity and unateness). For k-juntas, the functions used in the paper are illustrated in the following figure:
This figure shows how an input x in {0,1}n is evaluated by a function f used in the paper. Two disjoint subsets of randomly sampled variables, M and A, determine f(x) in the following way. Given x, one first interprets the variables in M as the index i of a function hi from a sequence of random functions, each of which depends only on a small subset of variables in A. Then f(x) is set to the value of hi evaluated on the relevant variables in the small subset of A (depicted by the four narrow shaded bands). By adjusting the size of A, f becomes either a k-junta or far from every k-junta, for some appropriate parameter k.
“Once we had the initial idea, the work went quickly; in less than a month, we had the result,” says Chen. “The surprise is that we could push it all the way to get the lower bound to match the upper bound, which has been known since 2008. This result has two implications. We know the exact number of queries needed for nonadaptive junta testing. And we know that adaptive algorithms not only do better than nonadaptive ones, they do a lot better, with k queries instead of k1.5.”
Adds Servedio, “Junta testing is one of the best-known problems in property testing of Boolean functions. It’s nice to have a thorough understanding of what is and isn’t possible for this basic problem. Hopefully the techniques we used for this result will find further applications for other problems in property testing and query complexity.”
In this video talk, Erik Waingarten gives more technical detail about the method used in the paper.
Posted 7/11/2017
– Linda Crane
Julia Hirschberg gives Fillmore Lecture at Linguistic Institute
Hirschberg’s subject was entrainment, the tendency of speakers to align their speaking style with that of a conversation partner. Joint work with Štefan Beňuš, Nishmar Cestero, Agustín Gravano, Rivka Levitan, Sarah Ita Levitan, Zhihua Xia.