Overview
Proximal junctional kyphosis (PJK) is a postoperative complication that occurs relatively frequently in the adult spinal deformity (ASD) population. The proximal junctional sagittal angle (PJA) is described as the Cobb angle formed by the caudal endplate of the upper instrumented vertebrae (UIV) and the cephalad endplate of the vertebral body two levels cephalad to the UIV. While PJK is defined differently throughout literature, the most frequently used criteria is a change in the PJA > 10˚ postoperatively in addition to an absolute value of the postoperative PJA > 10˚ .
Depending on the definition used and the population studied, PJK is most often reported to occur in 17% to 46% of patients. PJK requiring surgical intervention as a result of neurological deficits, pain, unacceptable kyphosis, or poor self-image is therefore termed proximal junctional failure (PJF).
We build a machine learning algorithm to identify novel metrics associated with PJK. Our algorithms are based on XGBoost, where we incorporate privileged information, from post-surgery data, through residuals calculated during each boosting round. Our model iteratively provides learned advice at each boosting round. We evaluate our model on PJK data from the Och Spine at NewYork-Presbyterian, along with synthetic and UCI datasets. Our proposed method consistently outperforms its vanilla counterpart on all datasets while maintaining its explainability prowess.
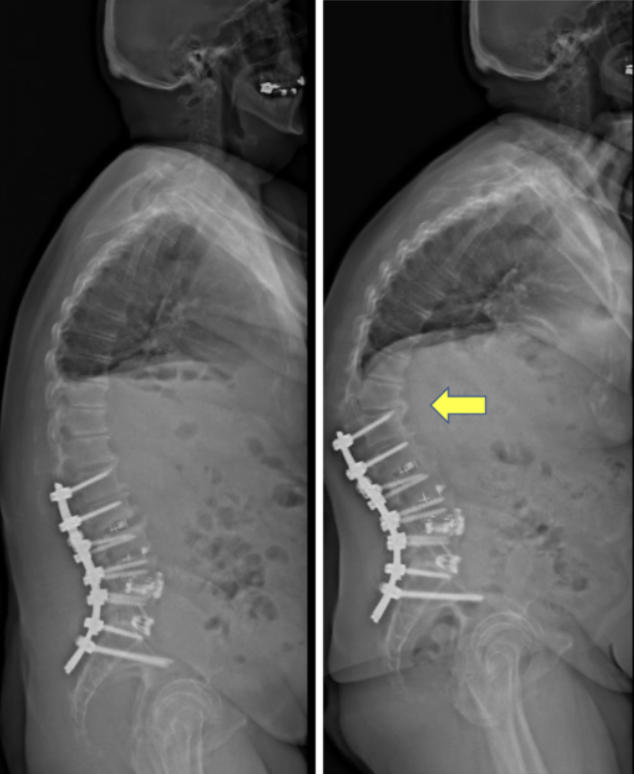
Members
Ansaf Salleb-Aouissi (co-PI)
Adam Lin
Rohith Ravindranath
Lawrence Lenke (co-PI)
Joseph Lombardi (co-PI)
Dr. Cole Morrissette
Dr. Mark Herbert
Dr. Yong Shen
Justin Reyes
Gabriella Greisberg
Past Members
Ritesh Baldva
Ammran H Mohamed
Amogh Inamdar