Overview
Necrotizing enterocolitis (NEC) is a common, life-threatening intestinal disease that affects approximately 10% of premature infants during the first few weeks after birth, usually in the setting of the neonatal ICU (NICU). Characterized by sudden-onset and progressive intestinal ischemia and necrosis, NEC is diagnosed in approximately 11,000 babies in the United States annually. The mortality rate associated with NEC is 15-30%. Furthermore, the risk of long-term neurodevelopmental consequences among NEC survivors is also significant.
Early diagnosis of NEC is thus critical to allow timely treatment and intervention, but there is currently no test, biomarker, or physiologic measure that predicts risk of disease onset. Instead, neonatal healthcare providers must closely monitor all premature infants for clinical signs of NEC. Common presenting symptoms are abdominal distention, vomiting, blood-tinged stools, and vital sign changes. Unfortunately, with no alternative to close clinical monitoring, many infants are diagnosed only after irreversible intestinal damage has already occurred.
Accurate NEC risk prediction challenging. Thus, methods are needed for early, precise bedside prediction of NEC in preterm patients. Central to our NEC predictive system is a neural network-based, semi-supervised machine learning strategy called multiple instance learning (MIL). Microbial taxa abundances from a single sample can almost never be described as “physiologic” or “pathologic” in isolation. Informative patterns can only be detected by comparing groups of affected and unaffected samples. However, multidimensional and highly variable comparisons can confound classical statistical approaches. Neural network-based MIL, on the other hand, is well-suited for identifying subtle, variable relationships among high-dimensionality, inherently unlabeled data such as from metagenomic microbiome sequencing. Our project aims to deliver a set of MIL-based tools for prediction of NEC from metagenomic sequencing data combined with optional clinical or demographic metadata.
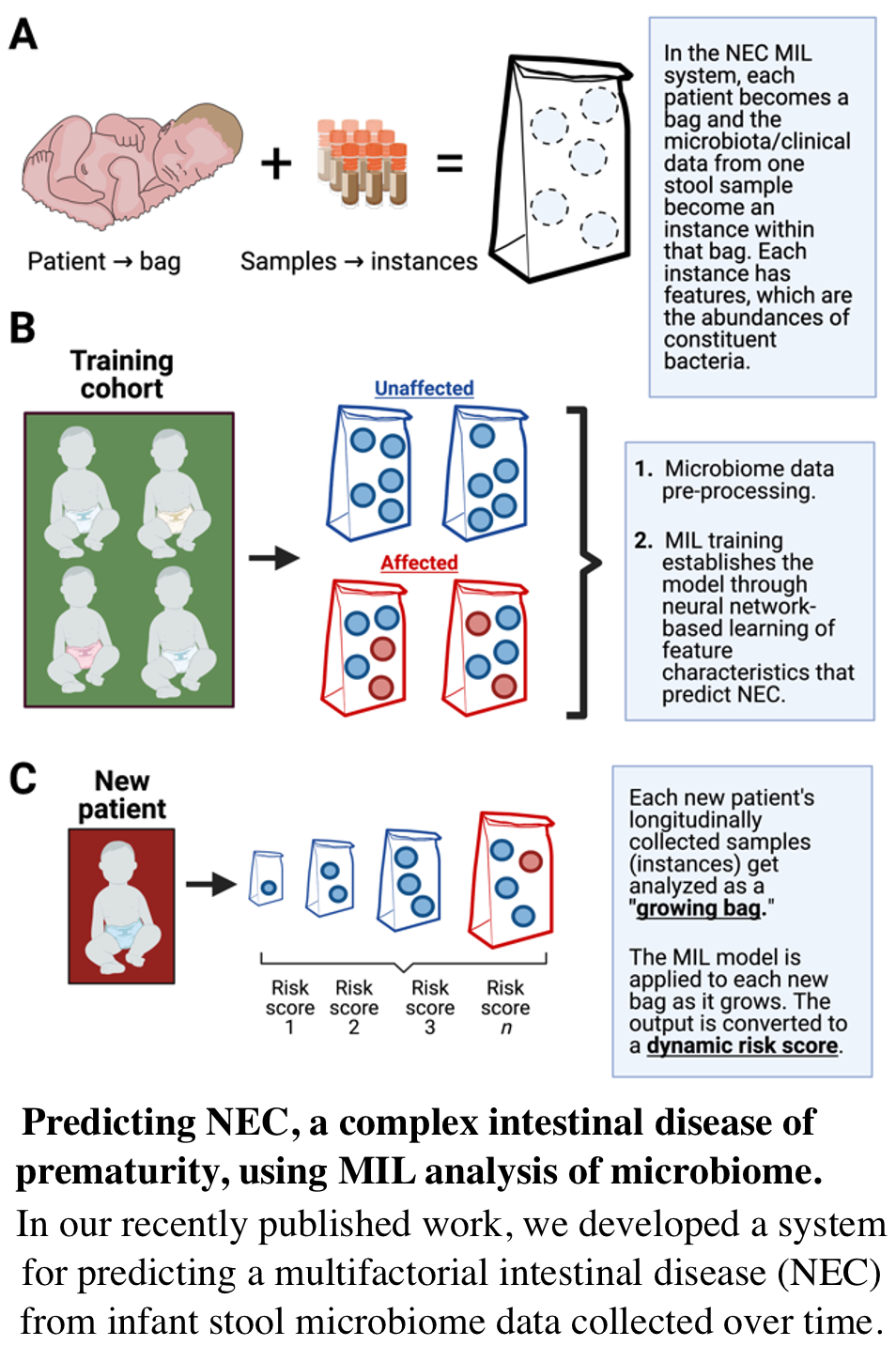
Members
Ansaf Salleb-Aouissi (co-PI)
Thomas Hooven (co-PI)
Adam Lin
Related Publications
Lin, Yun Chao, Ansaf Salleb-Aouissi, and Thomas A. Hooven. "Interpretable prediction of necrotizing enterocolitis from machine learning analysis of premature infant stool microbiota." BMC bioinformatics 23.1 (2022): 1-29.
Hooven, Thomas, Yun Chao Lin, and Ansaf Salleb-Aouissi. "Multiple instance learning for predicting necrotizing enterocolitis in premature infants using microbiome data." Proceedings of the ACM Conference on Health, Inference, and Learning. 2020.
Related Press Release
1- New Machine Learning Tool Predicts Devastating Intestinal Disease in Premature Infants
https://www.engineering.columbia.edu/news/machine-learning-premature-infants