Understanding Infant Colic
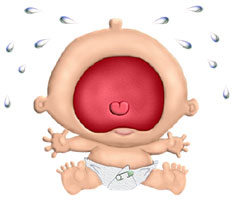
Infant colic is defined as persistent inconsolable crying in healthy babies between 2 weeks and 4 months of age, where the baby seems to be in great discomfort and is difficult to soothe. Colic is not a disease but a serious condition with medical and social consequences, yet its causes remain a mystery. Prevalence rates of excessive crying vary between definitions. Estimates of the num- ber of affected infants aged 0-6 months who cry three or more hours a day, three or more days a week, during three or more consecutive weeks for no clear cause (Wessel's criteria (Wessel et al., 1967)), range from 2% to 5% (Reijneveld et al., 2001).
Recent studies suggest that excessive crying in infancy has a small but significant adverse effect on cognitive development and mental health problems later in life [Wolke et al., 2009] and can lead to mother postpartum depression [Vik et al., 2009]. Finally, colic is costly for healthcare systems, due to various ineffective medications, doctor's office and emergency room visits, and even hospitalization. Treatment varies substantially from physician to physician. The medications doctors prescribe to treat colic or identify its causes often have side effects but don't provide a cure. The medical literature on colic is a mix of hypotheses to explain this mysterious condition. These include lack of bacteria in the intestines, reflux, lactose intolerance, maternal smoking, and parental depression, to cite a few.
We hypothesize that colic has discoverable root causes. We propose to conduct the first large scale study to tackle this problem through Machine Learning (ML) on a very large, high-dimensional database. We assume the underlying causes are complex, possibly a combination of variables, or distinct syndromes. Machine Learning is a powerful technology for constructing complex models in very high dimensional spaces that has proven useful in a wide range of arenas. Successful use of Machine Learning requires an understanding of the application domain, and where the data is not already in a form amenable to machine learning, it also depends on assembling comprehensive and trustworthy data.
This project is partially funded by a Research Initiatives in Science and Engineering (RISE) grant from the Columbia University Executive Vice President for Research and is being conducted under IRB-AAAF2852.
A RISE award allowed our project to establish a common ground among experts in machine learning, pediatric medicine, biomedical informatics, and natural language processing.
We have made substantial progress in assembling the data and store it in databases along with preprocessing and data cleaning.
Over 26 billion dollars are spent annually on the delivery and care of the 12-13% of infants who are born preterm in the United States. A crucial challenge is to understand the etiologies that drive preterm birth (PTB) and to identify women who are at risk through the different stages of pregnancy. Previous research has largely focused on individual factors correlated with PTB, including prior preterm birth, black race, multiple gestations and infection. Among these, clinicians rely most on prior preterm birth to identify women at risk who might benefit from treatments such as prenatal administration of progesterone. As a result, the 40% of pregnant women in the U.S. who will give birth for the first time (nullliparas) often go untreated. If it were possible to develop a reliable risk predictor of PTB for first-time mothers, it could substantially reduce the incidence of PTB and its consequences.
Our project combines three key preconditions for advancement in the arena of PTB: (a) large amounts of data stored in Electronic Health Records (EHRs) that offer an unprecedented statistical power complemented by, (b) several sets of well-curated, standardized data collected in the context of the Maternal-Fetal Medicine Units Network (MFMU) and that can be used to evaluate the data mining quality of EHRs and (c) the progress achieved in machine learning with the high-dimensional methodologies.
We propose to develop statistical models to assign risk levels during the pregnancies of nulliparas mothers that will predict those at most risk of spontaneous PTB. (Spontaneous PTB, meaning not induced, accounts for 70% of the cases.) We aim to develop a dynamic predictive model that takes into account different risk factors at different stages of pregnancy. We have assembled a database that brings together several types of EHRs for mothers and their babies.
This project is funded by the National Science Foundation
NSF.
More information about the RISE program:
http://researchinitiatives.columbia.edu/funding-resources/research-initiatives-science-and-engineering-rise
Risk Assessment of Spontaneous Preterm Birth
Picture of a 23 weeks preemie in an incubator (source: March of Dimes).
Please see the NSF project page here: here
This project is being conducted under IRB-AAAJ2054.
For more about prematurity, please refer to the recent report about preterm birth just released by the March of Dimes, World heath Organization and other entities.
http://www.who.int/pmnch/media/news/2012/201204_borntoosoon-report.pdf