Welcome!
Award Title:
EAGER: Collaborative Research: Advanced Machine Learning for Prediction of Preterm Birth
Acknowledgement:
This is a collaborative research project. This material is based upon work supported by the National Science Foundation under Grant No. IIS-1454855 (PI: Ansaf Salleb-Aouissi) and Grant No. IIS-1454814 (PI: Anita Raja). We are thankful to the National Science Foundation for supporting this project.
Disclaimer:
Any opinions, findings, and conclusions or recommendations expressed in this material are those of the author(s) and do not necessarily reflect the views of the National Science Foundation.
Last update: June 8, 2016.
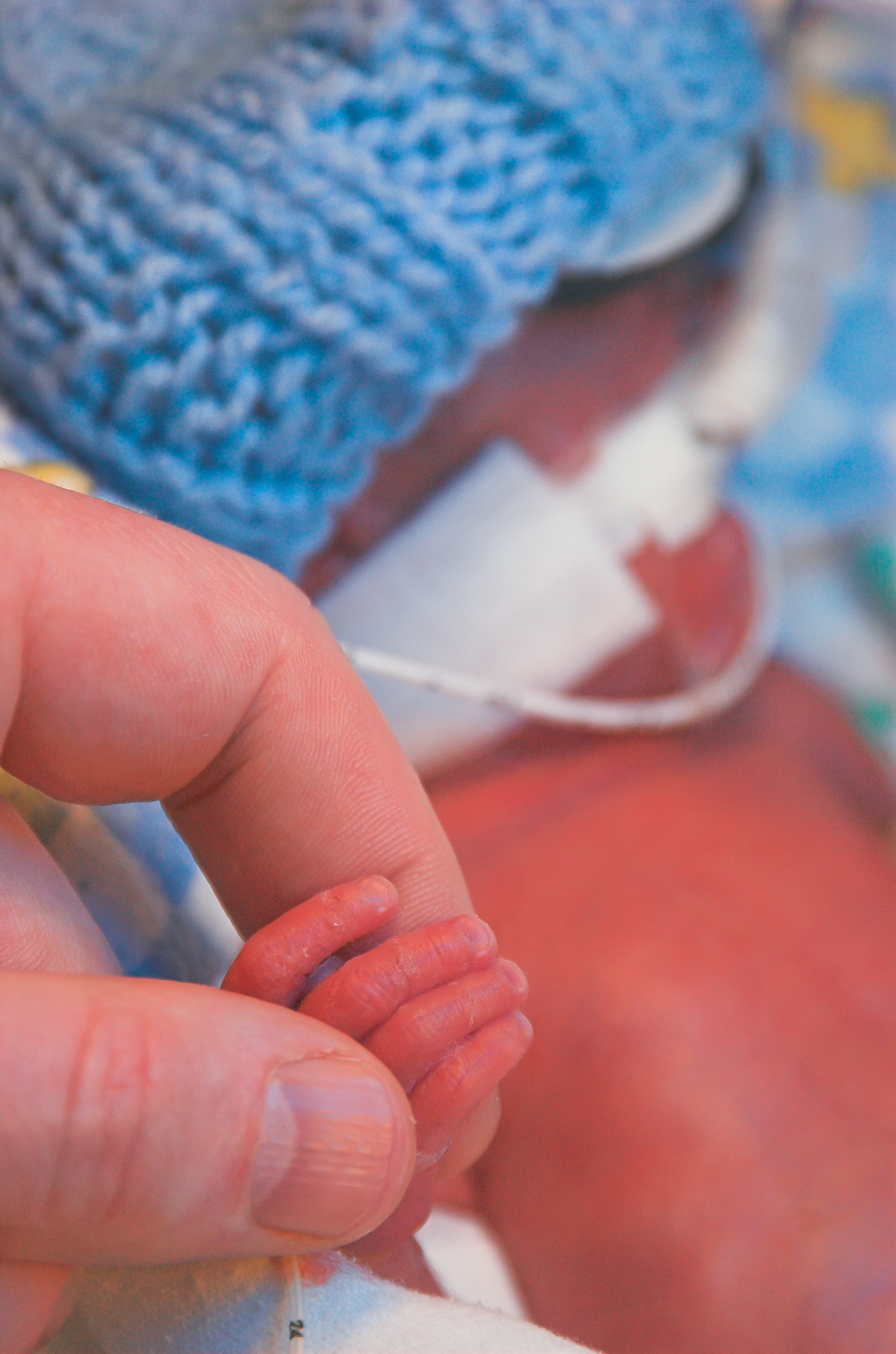
The United States spends over 26 billion dollars per annum on the delivery and care of the 12-13% of infants who are born preterm. As preterm birth (PTB) is a major public health problem with profound implications on society, there would be extreme value in being able to identify women at risk of preterm birth during the course of their pregnancy. Previous predictive approaches have been largely unsuccessful since they have focused on a limited number of well described risk factors known to be correlated with preterm birth (e.g. prior preterm birth, race, and infection) and less on combining multiple factors. The latter approach is necessary to understand the complex etiologies of preterm birth. While identifying individual PTB risk factors has brought insight into the problem and has led in some cases to successful treatments such as progesterone for women with a previous preterm birth, this has only a limited impact on the overall frequency since many at risk patients, such as first time mothers (nulliparous), go untreated. Today, there is still no widely tested prediction system that combines well-known PTB factors and is clinically useful. There is, however, a global awareness of the need to discover and integrate the complex etiologies of prematurity in order to predict women at risk. Significant efforts have been made in the last couple of decades to collect large curated datasets of pregnant women. Previous studies on these datasets used relatively straightforward biostatitistical methodologies such as relative risk assessments to measure associations between factors and PTB. However, risk factors are studied independently of each other, which does not account for the multifactorial complexity of PTB. This exploratory project aims to investigate the value of more advanced machine learning methods by simultaneously considering all the factors, to develop better predictive methods.
This project is being conducted under IRB-AAAJ2054
For more about prematurity, please refer to the recent report about preterm birth just released by the March of Dimes, World heath Organization and other entities.
http://www.who.int/pmnch/media/news/2012/201204_borntoosoon-report.pdf